Disclosure: At least one member of the Reflexivity Research team currently holds a position in Bittensor's token (TAO) at the time of writing/publishing. See the bottom of this write-up for more disclosures.
What is Bittensor?
Bittensor is a decentralized machine learning network built with the aim of creating a global neural network powered by a decentralized, incentive-driven infrastructure. Fundamentally, Bittensor allows AI models to contribute to a shared knowledge pool, receiving cryptocurrency rewards in return for their contributions to the network. The network’s primary goal is to incentivize the creation of intelligent, autonomous AI models by providing a decentralized environment where AI models can interact, learn, and improve in a trust-minimized way.
Bittensor reimagines the traditional model of centralized AI research by introducing decentralized and incentive-based learning. Instead of relying on a few large organizations or companies to provide AI models, Bittensor allows any participant to deploy their own AI model as a "node" in the network. These nodes communicate with each other, exchange information, and use a proof-of-learning mechanism to determine the quality and utility of the knowledge they share. This mechanism creates a competitive environment where the most valuable models receive more rewards, leading to an ecosystem of continuous improvement and innovation.
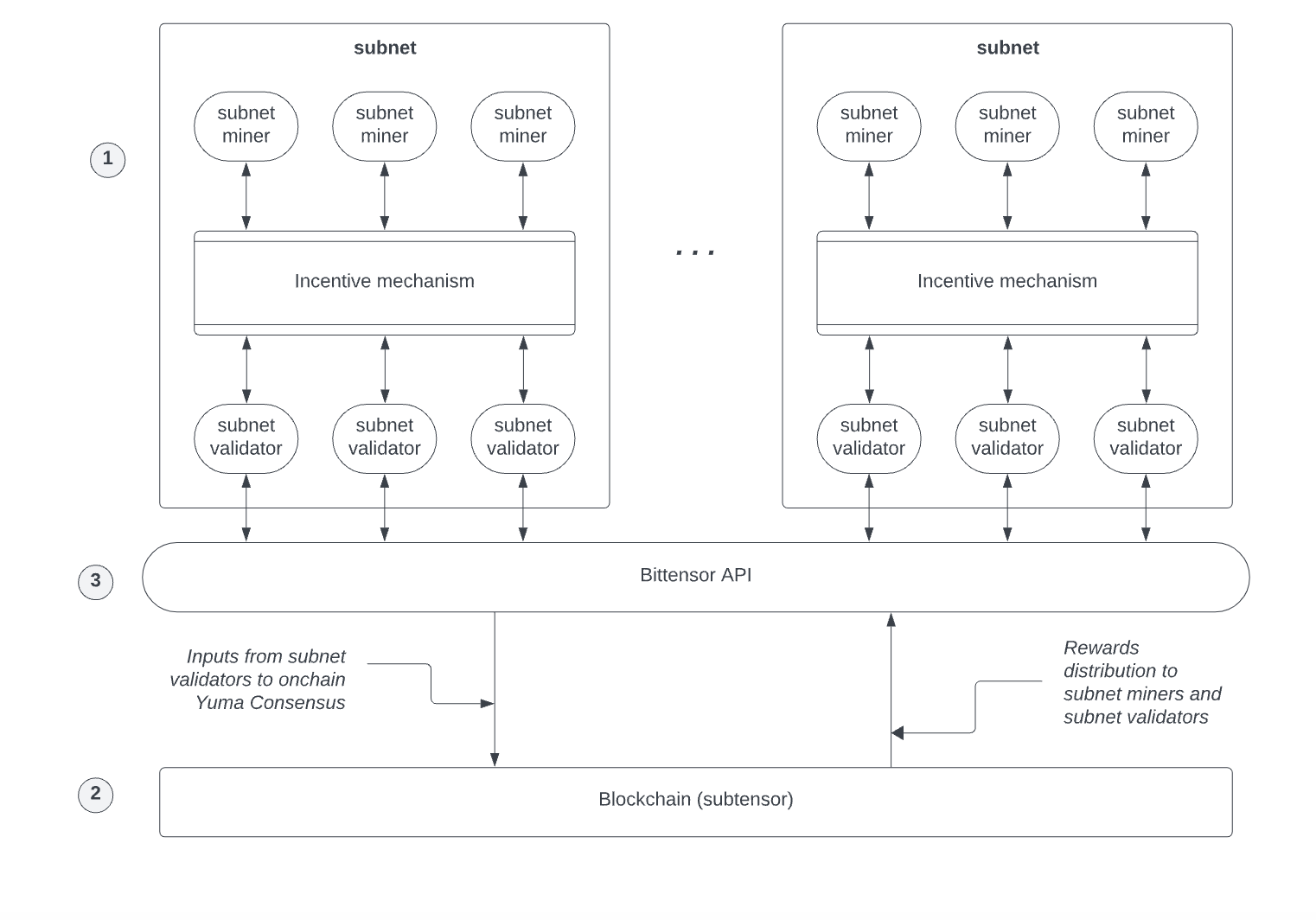
The incentive structure within Bittensor is designed around the network’s native cryptocurrency, TAO. Nodes earn TAO by providing useful information or training data to other nodes. The staking and tokenomics of TAO play a key role in driving the network’s dynamics, as participants stake TAO to access the network’s resources and interact with other nodes. The more valuable a node's contributions, the higher its rewards, thereby creating a self-sustaining cycle of knowledge exchange and refinement.
One of Bittensor’s fundamental goals is to develop an open and permissionless AI network where models of varying capabilities and purposes can collaborate to build more advanced intelligence. The decentralized nature of Bittensor ensures that the knowledge and data shared across the network are not controlled by any single entity, mitigating concerns around data monopolization and crafting a more equitable AI landscape. This contrasts with centralized AI development models, which are often hindered by privacy concerns, limited access to data, and the significant costs associated with model training.
The proof-of-learning mechanism is the heart of Bittensor’s incentive structure. This mechanism assesses the quality of the information provided by each node and its contribution to the overall network intelligence. When a node submits information, other nodes in the network can interact with it, providing feedback on its relevance and accuracy. The more a node's knowledge is utilized and validated by others, the more it earns in TAO. This dynamic encourages nodes to produce high-quality outputs and discourages spam or low-quality contributions, maintaining the integrity of the network.
In addition to the quality-driven incentive model, Bittensor aims to tackle some of the key challenges facing the AI community, such as scalability and interoperability. By creating an open network of interconnected models, Bittensor facilitates the exchange of knowledge across different AI domains, allowing for the rapid development of more versatile AI capabilities. This approach enables the network to evolve organically as new models and technologies are introduced, potentially leading to breakthroughs in AI research that would be more challenging to achieve in isolated, centralized environments.
A key innovation of Bittensor lies in its use of subnets. Bittensor's subnet architecture allows the network to partition nodes into different subgroups, each focusing on specific tasks or datasets. These subnets act as semi-independent neural networks within the larger Bittensor ecosystem, allowing for specialization and efficiency in knowledge sharing. For instance, one subnet might specialize in language processing, while another focuses on image recognition. This compartmentalization not only helps optimize the network's resources but also encourages the development of diverse AI models tailored to specific use cases.
Subnets in Bittensor are designed to be autonomous yet interconnected, enabling nodes within a subnet to communicate and share knowledge relevant to their specialization. Each subnet operates under a shared protocol that defines the rules for interaction, consensus, and reward distribution within that subnet. This structure allows Bittensor to scale effectively by avoiding a one-size-fits-all approach to data processing and knowledge exchange. By dividing the network into focused subnets, Bittensor can handle a broader range of tasks more efficiently, making it a more flexible and powerful platform for AI research and development.
Bittensor’s subnet system introduces a layered approach to the network’s overall intelligence. Within each subnet, nodes work together to improve their specialized knowledge, while still being part of the larger Bittensor network. Nodes in different subnets can cross-communicate, allowing for an exchange of information across different domains of AI. This cross-pollination of knowledge ensures that insights from one subnet can inform and enhance the performance of models in another, creating a dynamic, interconnected web of learning.
One of the key aspects of Bittensor’s subnets is their ability to be permissionless and self-organizing. Any participant can create or join a subnet, and the network dynamically adjusts to the contributions of each node. This open structure fosters a competitive yet collaborative environment, where nodes are incentivized to optimize their contributions to their subnet to maximize their rewards. Additionally, the subnet system allows for rapid experimentation, as developers can launch new subnets to explore novel AI models or training methods without disrupting the entire network.
Each subnet operates under a governance model that is distinct yet aligned with the broader Bittensor network. This model involves consensus mechanisms to validate the contributions of nodes within the subnet and determine the allocation of rewards. These mechanisms ensure that subnets remain efficient and that the knowledge exchanged within them maintains a high standard of quality. Subnet governance also allows for the fine-tuning of the proof-of-learning algorithm to best suit the specific needs and goals of each subnet, enhancing the flexibility and adaptability of the overall network.
The future vision for Bittensor involves decentralizing AI by expanding its network and subnet architecture. The project aims to grow the network's diversity by encouraging the development of new subnets tailored to emerging AI domains, such as natural language processing (NLP), computer vision, reinforcement learning, and more. By increasing the number and variety of subnets, Bittensor hopes to create a vast, decentralized intelligence network where AI models can continually learn, adapt, and evolve through shared knowledge.
Additionally, the TAO token will continue to play a central role in driving the network's growth and participation. Future plans include refining the tokenomics to continue incentivizing high-quality contributions and participation in governance. The staking mechanism might also evolve to support more complex interactions, such as allowing participants to delegate their stake to specific subnets or nodes they believe are most valuable. This will deepen the engagement within the community and enhance the network’s collective intelligence.
Bittensor's roadmap includes expanding its interoperability with other decentralized AI and blockchain projects, facilitating the seamless integration of its knowledge network into broader Web3 ecosystems. This could involve developing bridges to other blockchain platforms, enabling the use of Bittensor’s AI capabilities across decentralized applications (dApps). By positioning itself as a core AI infrastructure within the decentralized world, Bittensor aims to become a key player in shaping the future of AI development.
Bittensor represents a significant leap forward in decentralized AI research. By combining incentivized knowledge sharing, a modular subnet architecture, and a robust proof-of-learning mechanism, it provides a platform where AI models can collaborate, compete, and evolve without the constraints of centralized control. The subnet system enhances the network's scalability and specialization, setting the stage for a new era of AI development driven by decentralized intelligence.
Why is Bittensor necessary?
The current state of artificial intelligence and machine learning is marked by significant centralization, with control and development often limited to large corporations and research institutions. This centralization results in several challenges: the monopolization of valuable datasets, limited access to advanced AI tools for smaller entities, and the potential for bias in model training. Additionally, the closed nature of many AI platforms means that most of the data used for training remains siloed, exacerbating privacy concerns and limiting the diversity of perspectives integrated into AI models. In this landscape, Bittensor presents an alternative approach, leveraging the principles of decentralization and blockchain technology to create an open, incentivized AI network.
Blockchain technology, particularly when combined with decentralized protocols like Bittensor, offers a new paradigm for AI development. Bittensor's network operates under a permissionless framework, allowing anyone to contribute to and benefit from its AI models. This open-access model contrasts sharply with traditional AI research, where access is often restricted to those with extensive resources or institutional support. By incorporating blockchain, Bittensor provides a trust-minimized environment where AI models, or nodes, can interact and exchange knowledge transparently, without relying on a central authority. This decentralized setup is crucial for managing issues such as data privacy, ownership, and access in an equitable manner.
The role of crypto-economics in Bittensor's architecture cannot be overstated. Traditional AI research is usually funded by grants, commercial interests, or internal budgets, which inherently limits the scope and direction of AI projects. In contrast, Bittensor introduces a self-sustaining incentive mechanism powered by its native token, TAO. This system incentivizes nodes to share valuable insights and continuously improve their models. By aligning economic incentives with knowledge sharing, Bittensor creates a collaborative ecosystem where nodes are motivated to contribute high-quality data and intelligence. This is a marked shift from traditional AI, turning research into a competitive yet symbiotic process that ensures continuous model refinement.
A critical aspect of Bittensor's necessity lies in its ability to address the bias and diversity limitations present in current AI models. Centralized AI systems often train on datasets curated by specific entities, leading to potential biases in their outputs. Bittensor, on the other hand, allows nodes worldwide to provide diverse data inputs, contributing to more comprehensive and balanced AI models. This decentralized approach not only democratizes data access but also results in more robust and reliable AI outputs, as the models are continuously validated and improved by a wide range of participants in the network.
Additionally, the network’s proof-of-learning mechanism ensures that rewards are distributed based on the quality of contributions. This framework addresses one of the major issues in AI today: the lack of a standardized, open evaluation process for determining the value of AI outputs. In traditional settings, the quality of AI models is often judged by proprietary benchmarks, limiting the scope for open collaboration. Bittensor's proof-of-learning system offers a transparent, community-driven approach to evaluating contributions, thereby promoting merit-based rewards. This method fosters a more innovative environment, where nodes are encouraged to explore new techniques, optimize their models, and share their findings with the network.
Bittensor’s subnet architecture serves to enhance its sustainability and utility by allowing specialized AI models to collaborate within specific domains. Subnets focus on tasks ranging from natural language processing to computer vision, providing an efficient and dynamic learning environment. In traditional AI development, specialization often occurs in isolated silos, limiting the opportunity for cross-domain knowledge transfer. Bittensor’s interconnected subnets overcome this barrier, creating an ecosystem where different AI models can learn from each other’s advancements. For example, improvements in one subnet’s language processing capabilities can inform models in other subnets, facilitating a holistic growth of network intelligence.
By utilizing blockchain's transparency and security, Bittensor also tackles the issue of data integrity and trust. Traditional AI models often require users to trust the central entity managing the data and the model’s training process, which can lead to concerns over data misuse or manipulation. Bittensor ensures that all transactions and interactions are recorded on a public ledger, allowing participants to verify the authenticity and provenance of the information shared within the network. This transparency builds trust among contributors and end-users, enhancing the network's credibility and long-term viability.
Bittensor’s incentive mechanisms also address a broader issue within the AI and crypto intersection: the need for sustainable funding models that promote continuous innovation. In many AI projects, funding is a one-time effort that relies on grants, investments, or corporate backing, which may lead to short-lived projects with limited development. Bittensor’s tokenized incentive structure provides a continuous stream of rewards, motivating ongoing contributions and improvements. This not only sustains the network but also drives the rapid evolution of AI models, as participants are constantly rewarded for meaningful, high-quality contributions.
Bittensor's use of decentralized AI aligns with the growing demand for AI ethics and data privacy. Traditional AI development often involves the collection and processing of vast amounts of user data, raising concerns about data privacy and misuse. Bittensor’s network mitigates these risks by decentralizing data ownership, allowing contributors to maintain control over their inputs. Additionally, the network's built-in encryption and privacy measures provide a secure environment for data exchange, reinforcing Bittensor's commitment to ethical AI practices.
In the broader context of AI and crypto convergence, Bittensor exemplifies how decentralized AI can revolutionize both industries. It offers a solution to the centralization and exclusivity that currently hinder AI's full potential by providing an open, collaborative platform powered by blockchain and crypto-economic principles. By doing so, Bittensor not only advances AI research but also demonstrates the power of blockchain to enable new models of innovation, equity, and sustainability in the digital economy. This convergence could be a defining factor in the next wave of AI and blockchain applications, positioning Bittensor as a cornerstone of the decentralized intelligence future.
How do Bittensor’s subnets work?
Bittensor's subnet system forms the backbone of its decentralized AI network, allowing for the creation of specialized environments where different AI models can interact, train, and improve autonomously. Unlike traditional machine learning or artificial intelligence development, which often relies on centralized servers and datasets, Bittensor’s subnet architecture introduces a modular and decentralized approach to AI training. This system allows AI models to exist in subnets tailored to specific tasks or datasets, promoting diversity, specialization, and scalability within the network.
Each subnet in Bittensor acts as a semi-independent neural network focused on a particular type of intelligence or data processing. Nodes (AI models) within these subnets work together by exchanging knowledge in the form of input-output pairs, learning from one another, and building a shared understanding of their specific domain. The subnet system is underpinned by Bittensor's proof-of-learning mechanism, which enables nodes to evaluate the quality and relevance of the knowledge shared within the subnet. This fosters a competitive environment where nodes are incentivized to provide valuable, high-quality contributions to earn rewards in TAO tokens.
In traditional AI/ML development, models are trained on static datasets in centralized environments, often requiring extensive computational resources and data privacy considerations. By contrast, Bittensor's subnets offer a dynamic, distributed training environment where nodes can constantly adapt and improve based on real-time interactions with other nodes in the subnet. This decentralized approach addresses several limitations of traditional AI development. For one, it eliminates the need for centralized data ownership, as knowledge is continually shared and validated within the subnets. Additionally, it provides a more scalable method for training models, as the network can easily expand by adding more nodes and subnets, each contributing to the overall intelligence of the ecosystem.
One of the key features of Bittensor’s subnets is their autonomy. Subnets operate independently but remain interconnected with the broader Bittensor network. They function according to specific protocols that dictate how nodes interact, reach consensus, and allocate rewards. This autonomous operation allows each subnet to optimize for its specific tasks, such as natural language processing, image recognition, or reinforcement learning. Despite this autonomy, subnets can still communicate with one another, allowing cross-pollination of knowledge and facilitating a more diverse and robust AI network.
The permissionless nature of Bittensor's subnets is another significant departure from traditional AI models. In centralized AI development, only specific institutions or companies with access to vast resources can contribute to model training and improvement. Bittensor’s subnet system allows anyone to create or join a subnet, regardless of their computational resources. This democratizes AI development and encourages a wider range of contributions, potentially leading to more innovative models and techniques emerging within the network.
The subnet system also introduces a layered approach to Bittensor’s overall intelligence. Each subnet focuses on a specialized task, and nodes within that subnet collaborate to enhance their understanding of their specific domain. The modular design of these subnets means that they can evolve and adapt as the network grows, ensuring that Bittensor remains a cutting-edge platform for decentralized AI. The self-organizing aspect of these subnets means that they can dynamically adjust to changes in participation, knowledge demand, and the overall network environment, maintaining a high level of efficiency and scalability.
Each of these subnets operates independently but contributes to the overall intelligence of the Bittensor network. They are built to handle specific types of data and learning tasks, allowing for specialization that is difficult to achieve in traditional, monolithic AI systems. The cross-communication between subnets enables knowledge gained in one domain to inform others. For example, improvements in natural language processing in Subnet X could enhance the language understanding capabilities of nodes in other subnets, such as those dealing with sentiment analysis in image recognition.
By implementing a proof-of-learning mechanism within each subnet, Bittensor ensures that only high-quality contributions are rewarded, maintaining the integrity and utility of the network. This is a stark contrast to traditional AI systems, where model performance is often dependent on the quality and size of centralized datasets. In Bittensor’s subnets, the decentralized validation process helps to continuously refine and improve the models, promoting a self-sustaining cycle of knowledge sharing and AI evolution.
Exploring the subnet ecosystem
There are over 49 subnets listed on taopill, but this section will focus on a handful recently highlighted by Sami Kassab in an August report. This doesn’t mean every subnet isn’t worth exploring, but rather it’s better to focus on some recent developments for the network and what teams are working to solve.
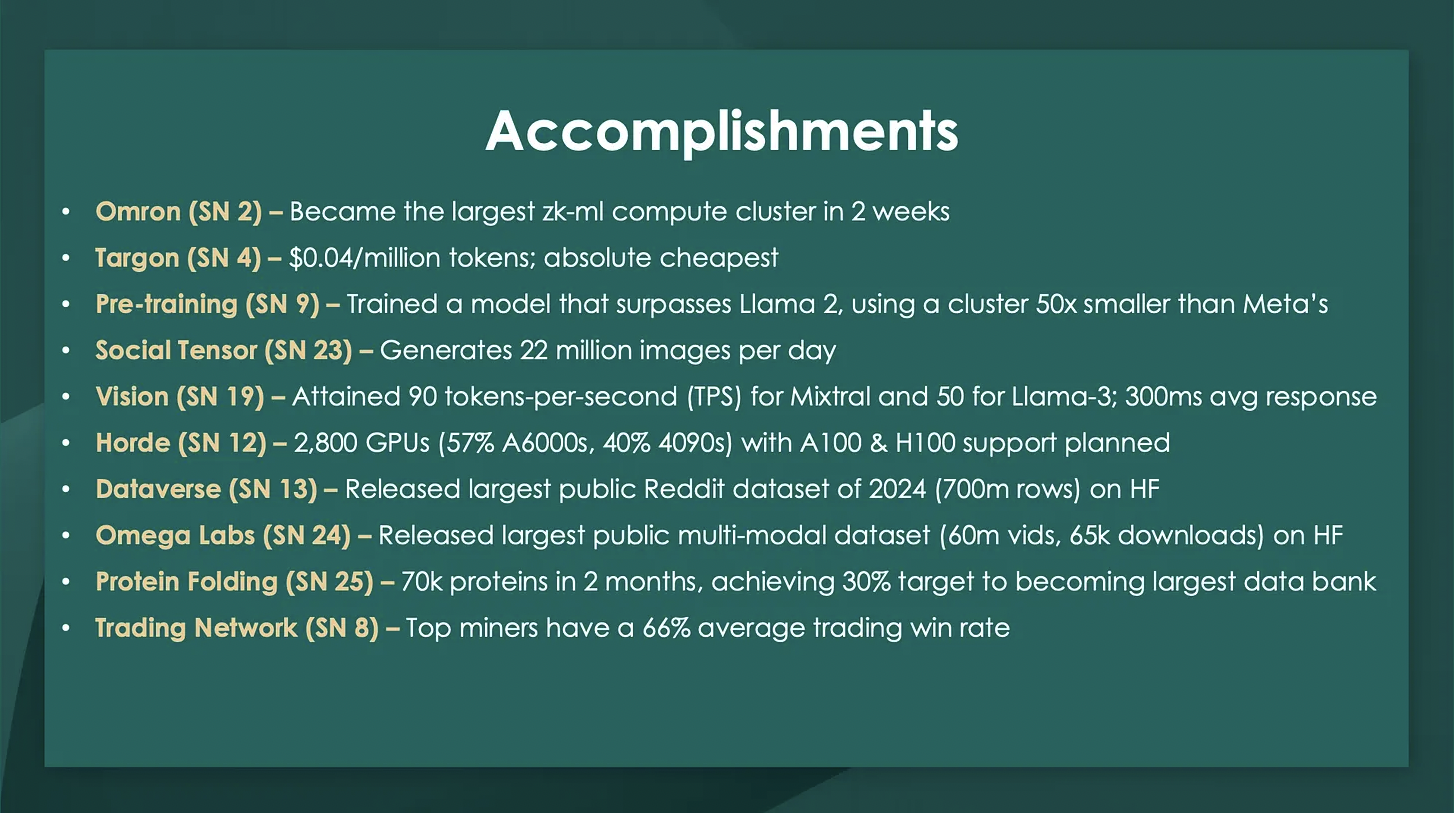
Omron (SN 2) quickly grew to become the largest zero-knowledge machine learning compute cluster in just two weeks. This achievement highlights Bittensor's capability to facilitate rapid development and scaling in decentralized AI.
Zero-knowledge proofs play a critical role in processing data securely without revealing the actual data, addressing privacy concerns often present in centralized AI systems. The success of Omron showcases Bittensor's potential to enable advanced AI solutions while preserving privacy, an essential feature in decentralized networks where data ownership and confidentiality are paramount.
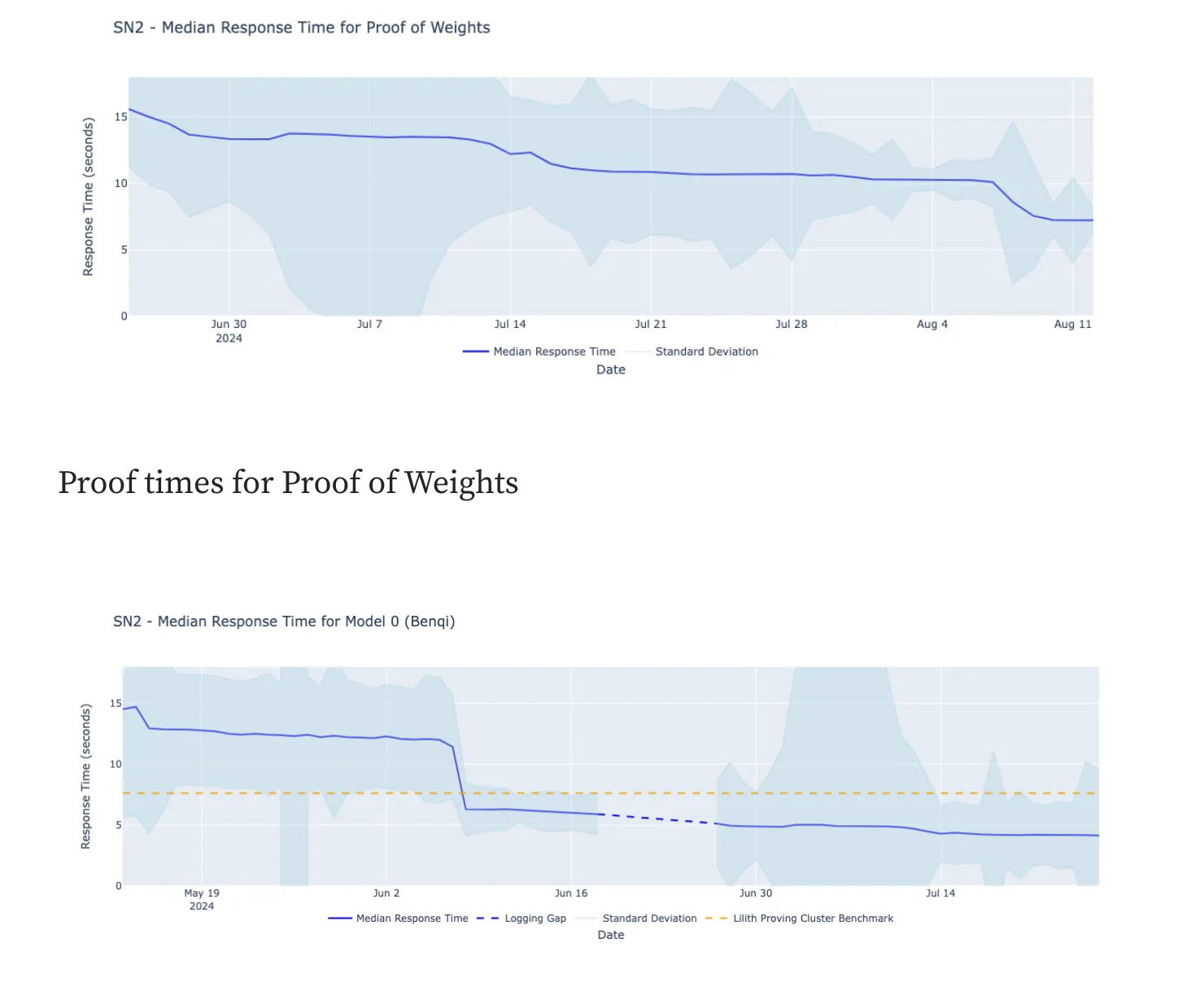
If you’re curious about Omron and want to dig deeper, the team wrote an excellent summary in late August providing their roadmap, current progress, and where they’re headed.
Targon (SN 4) stands out due to its extremely cost-effective computation, charging just $0.04 per million tokens. This approach drastically reduces the economic barriers typically associated with AI research and development, making it accessible to smaller developers, researchers, and independent contributors. In the context of Bittensor, this low-cost model democratizes access to AI capabilities, promoting diversity within the network. By creating an inclusive environment, Targon helps Bittensor attract a broader range of participants, driving the network toward its goal of creating a rich, decentralized AI ecosystem.
Macrocosmos is running a pre-training (SN 9) subnet, and has achieved a remarkable milestone by training a model that surpasses Meta's LLaMA 2, using a compute cluster 50 times smaller than Meta's.
This accomplishment is significant, as it demonstrates that high-performance AI models can be developed in a decentralized network without the need for vast computational resources typical of large tech companies.

By achieving competitive results with a more resource-efficient setup, SN 9 exemplifies how Bittensor's distributed architecture can produce powerful AI solutions. Macrocosmos believes that as time goes on and it becomes increasingly difficult for the average research lab to acquire sufficient amounts of compute, the prospect of decentralized training is only going to further accelerate. This approach aligns with Bittensor's mission to challenge the centralized AI development paradigm and encourage a more open, collaborative landscape for machine learning.
Social Tensor (SN 23) generates an impressive 22 million images daily, positioning itself as a key player in image-based AI models and social media-related tasks. The sheer volume and diversity of images processed within this subnet enrich the network’s capabilities, providing high-quality data for other nodes to leverage. In a decentralized AI ecosystem, access to a wide range of visual data is invaluable for developing models in computer vision, automated content moderation, and real-time image recognition. The output of Social Tensor not only contributes to Bittensor's collective knowledge but also demonstrates how specialized subnets can address complex, data-intensive applications.
Vision (SN 19) showcases Bittensor’s prowess in real-time data processing by achieving 90 tokens-per-second for Mixtral and 50 tokens-per-second for LLaMA-3, with an average response time of just 300ms. This level of performance is crucial for applications requiring immediate feedback, such as autonomous systems, live content filtering, and interactive AI platforms.
By providing rapid and efficient token processing, Vision significantly boosts the network's overall utility, catering to real-world demands for AI that operates in dynamic environments. Its success emphasizes the potential of decentralized AI to handle high-throughput, real-time tasks effectively.
Horde (SN 12) commands an extensive array of 2,800 GPUs, comprising 57% A6000s, 40% A4090s, and additional support from A100 and H100 GPUs. The sheer computational power of this subnet enables it to tackle complex machine learning models that require significant processing resources, such as deep neural networks and advanced reinforcement learning tasks.
Horde's contribution to Bittensor is invaluable, as it lays the foundation for scalability and the capacity to support resource-intensive applications. By pooling computational power from diverse sources, Horde reinforces the network's ability to train sophisticated AI models in a decentralized manner.
Dataverse (SN 13) has released the largest public Reddit dataset of 2024, with 700 million rows accessible on the Hugging Face platform. This dataset serves as a rich repository for NLP and sentiment analysis, enabling nodes within Bittensor to train models capable of understanding and interpreting human language with greater nuance.
In decentralized AI research, publicly available datasets like those provided by Dataverse are vital for incentivizing innovation and model accuracy. By contributing such a comprehensive dataset, Dataverse supports the network's goal of creating open-access AI resources for developers worldwide. For more info on SN 13 and how they’re looking to expand data scraping for Bittensor, their blog is an excellent resource.
Omega Labs (SN 24) brings a multi-modal approach to Bittensor by releasing a dataset containing 60 million videos, with 65,000 downloads on Hugging Face. The diversity and scale of this dataset support the training of models that can process and understand both audio and visual inputs, making it a valuable resource for applications in video analysis, complex audio-visual interactions, and augmented reality.
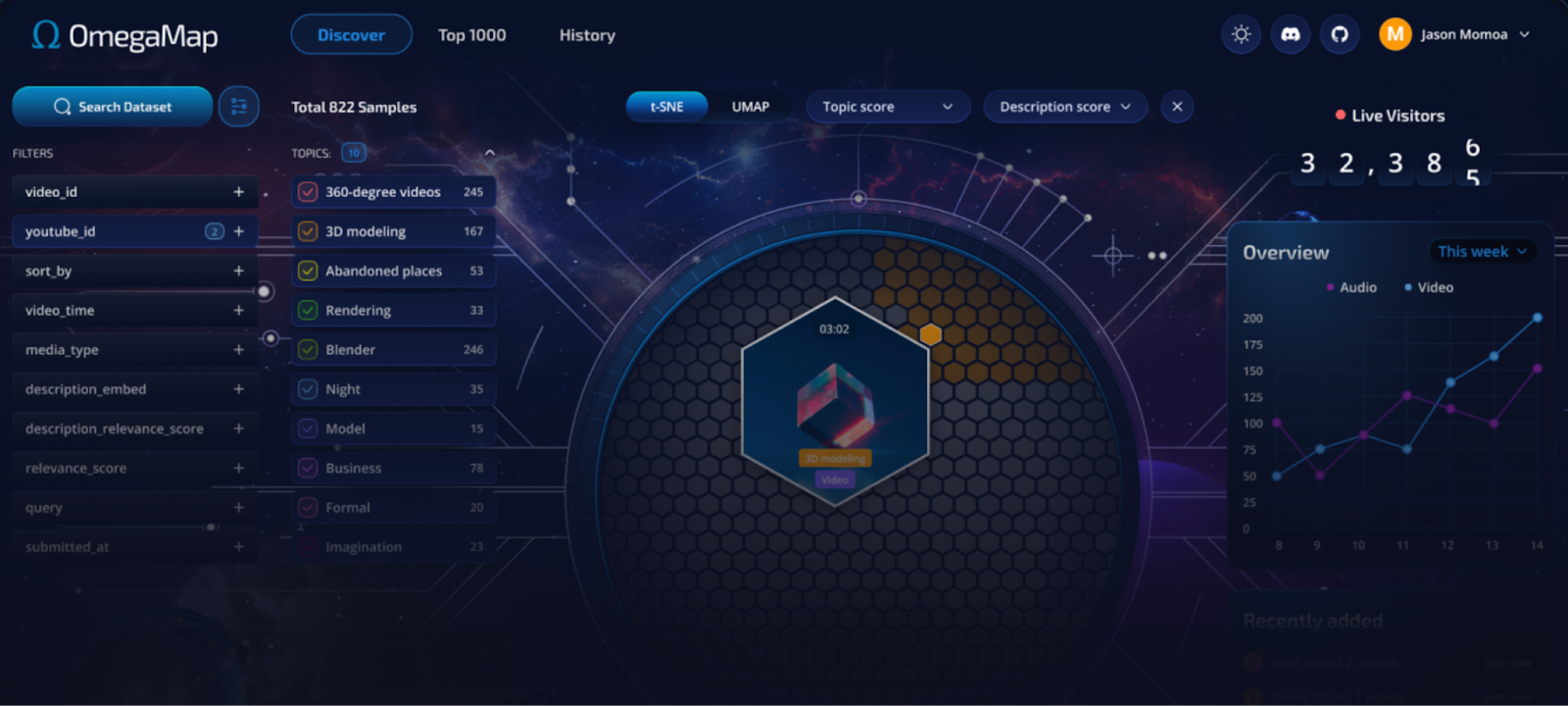
In a decentralized network, multi-modal AI capabilities are critical, as they enable nodes to handle a wide range of data types and use cases. Omega Labs' contribution broadens the scope of Bittensor's ecosystem, facilitating cross-domain learning and enhancing the network's adaptability.
Protein Folding (SN 25) has made significant strides in biological data processing by examining 70,000 proteins in just two months, achieving 30% of its target toward becoming the largest protein data bank in the network. This focus on protein folding has profound implications for biotechnology, pharmaceuticals, and healthcare applications. The team recently posted their plans for scaling SN 25 and what the future of biology on Bittensor might resemble - it’s worth a read.

By providing a decentralized platform for protein analysis, this subnet allows researchers to collaborate on complex biological challenges, potentially accelerating discoveries in drug development and molecular biology. Protein Folding's work illustrates how decentralized AI can impact scientific research domains, leveraging shared computational power and knowledge to solve intricate problems.
Trading Network (SN 8) demonstrates the potential of decentralized AI in financial markets, with top miners achieving an average trading win rate of 66%. This subnet specializes in market analysis and algorithmic trading, utilizing AI models to interpret market trends and execute trades. The success of the Trading Network shows how decentralized intelligence can outperform traditional, centralized trading algorithms, offering more dynamic and responsive market strategies. This subnet not only solidifies Bittensor's relevance in finance but also exemplifies how AI can thrive in decentralized environments, providing valuable insights and strategies in an ever-changing market landscape.
As a whole, these subnets contribute to Bittensor's goal of creating a versatile, scalable AI ecosystem. Their individual successes and focus areas—ranging from natural language processing and computer vision to medical research and financial analysis—enrich the network's collective intelligence.
The diversity of these subnets fosters cross-domain learning, where advancements in one area can inform and enhance models in others. Together, they embody Bittensor's vision of a decentralized, permissionless AI landscape that supports innovation, collaboration, and the continuous evolution of artificial intelligence.
Bittensor and some of its competitors
Bittensor, Akash, and Gensyn are protocols in the decentralized AI and compute space, each approaching different aspects of AI development and deployment. While they target various verticals within the AI and blockchain intersection, they share the common goal of decentralizing computational resources and AI training. However, their strategies, ecosystems, and long-term visions vary significantly, allowing them to coexist while occasionally overlapping in functionality and competition.
Bittensor is primarily focused on building a decentralized, collaborative AI network, emphasizing incentivized knowledge sharing among AI models. What sets Bittensor apart is its ambition to create a global AI network where any participant can contribute to and benefit from the shared pool of intelligence.
By incentivizing high-quality contributions using its native token, TAO, Bittensor has constructed a unique economic model that drives continuous innovation and model refinement within its ecosystem. Its vision goes beyond just training or utilizing AI; it’s about constructing a self-sustaining, decentralized AI society.
On the other hand, Akash Network operates as a decentralized compute marketplace that connects users needing computational resources with providers willing to rent out their unused computing power. It positions itself as a decentralized alternative to traditional cloud providers like AWS and Google Cloud, offering a permissionless and open marketplace for developers to deploy their applications.

Akash does not directly focus on AI or ML but provides the infrastructure necessary for computationally heavy tasks, including AI training. Its strength lies in its flexibility and cost-effectiveness, as users can deploy their projects on an open, peer-to-peer network without being bound to a single provider. This makes Akash a valuable resource for AI projects, potentially including those operating within the Bittensor network, which often require substantial computational resources to train and run models.
Gensyn is more directly aligned with the decentralized AI sector but targets a different aspect: decentralized training. Gensyn offers a protocol designed to facilitate AI model training on a global scale by connecting developers with a decentralized pool of compute resources. It aims to create an environment where AI models can be trained across a distributed network, using cryptographic verification to ensure that training tasks are completed accurately. The team building Gensyn believes that decentralized training is the only path forward - it’s in humanity’s best interest to ensure centralized AI and ML research labs don’t accumulate superintelligence before the rest of us even have a chance to catch up.
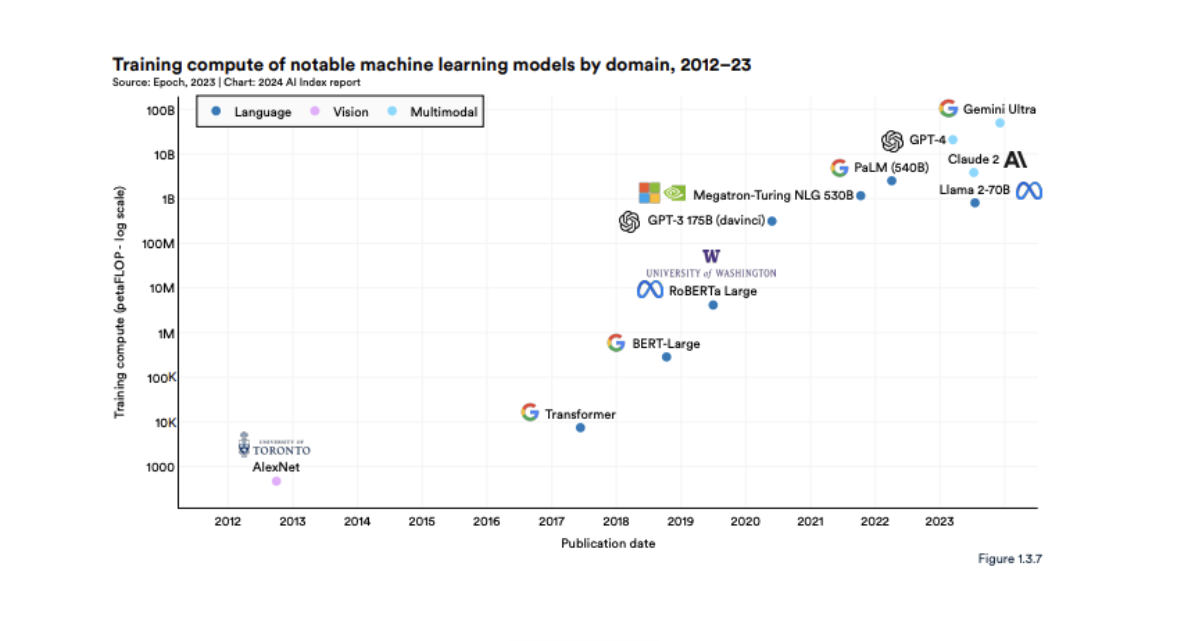
By utilizing blockchain technology to verify and reward contributions to model training, Gensyn focuses on decentralizing one of the most resource-intensive parts of the AI development process: the training phase. Unlike Bittensor, which aims to incentivize both knowledge exchange and model improvement, Gensyn’s primary goal is to create an open marketplace for decentralized model training.
While these three protocols operate in overlapping spaces, their differences in focus create potential for coexistence over longer time horizons. Akash can serve as the computational backbone for projects requiring flexible, decentralized infrastructure, not just for AI but for a broad range of applications. AI-focused projects, including those running on Bittensor or Gensyn, can utilize Akash’s decentralized compute marketplace to access the necessary resources for model training and deployment.
Bittensor and Gensyn, though both operating within the Decentralized AI domain, differ in their major objectives: Bittensor centers on the collaborative exchange of intelligence and the growth of a decentralized AI network, while Gensyn zeroes in on decentralizing AI training, providing developers access to global compute resources to build and refine their models.
Bittensor’s lofty ambitions and unique subnet architecture set it apart, potentially positioning it to compete directly with these protocols in the future. By enabling subnets that can specialize in a variety of tasks, Bittensor can expand into multiple verticals. For instance, subnets focusing on decentralized compute could indirectly compete with Akash by providing an incentive-driven platform for hosting computational workloads, particularly those related to AI. Additionally, Bittensor’s subnet ecosystem could incorporate decentralized model training services akin to Gensyn's, potentially establishing nodes that focus on executing and verifying training tasks within its incentivized framework.
The flexibility of Bittensor’s subnet system means that it could, in theory, integrate aspects of both Akash's compute marketplace and Gensyn’s training verification process into its own network. By doing so, Bittensor could offer a more comprehensive solution, covering the entire lifecycle of AI development, from training and model sharing to deployment and continuous learning. Its collaborative, decentralized nature makes it adaptable to various use cases, allowing it to expand into sectors that Akash and Gensyn currently target. However, for Bittensor to effectively compete in these areas, it would need to maintain the integrity of its incentive mechanisms and ensure that the subnet ecosystem can handle diverse computational tasks efficiently.
While Bittensor, Akash, and Gensyn share common ground in promoting decentralized AI and computational resources, they approach the problem from different angles. Bittensor focuses on building a decentralized AI network that thrives on collaborative intelligence and shared knowledge, leveraging its token-driven incentives to fuel ongoing model evolution. Akash provides the underlying infrastructure for decentralized compute, offering a flexible and cost-effective alternative to centralized cloud providers. Meanwhile, Gensyn zeroes in on the AI training phase, creating a protocol for verifying and rewarding decentralized model training tasks. Together, these protocols contribute to a more open, decentralized AI landscape, with the potential for symbiotic relationships. As Bittensor’s ecosystem evolves, its ability to adapt its subnets to various tasks may allow it to encroach on the domains of both Akash and Gensyn, ultimately competing across multiple verticals in the decentralized AI ecosystem.
Future plans for the network
Bittensor is on the path to becoming a comprehensive, decentralized AI network capable of hosting and evolving a wide variety of AI models through its expansive subnet ecosystem. With its innovative proof-of-learning incentive model and flexible subnet architecture, Bittensor has already set the stage for collaborative, real-time AI development. Moving forward, its primary focus is on scaling this collaborative ecosystem, attracting more participants, and refining its reward mechanisms to ensure that high-quality contributions are consistently incentivized.
One of Bittensor’s goals is to build out its subnet ecosystem, allowing for specialization and the development of models across different AI verticals. By doing so, Bittensor hopes to create a dynamic environment where AI models can evolve and adapt to various tasks, from natural language processing to computer vision and reinforcement learning. The network's permissionless structure will continue to foster inclusivity, attracting developers, researchers, and data scientists who can contribute their expertise and computational resources to grow the network. This approach not only enriches the models within Bittensor but also sets a foundation for tackling more complex and diverse AI challenges in the future.
In terms of competition, Bittensor plans to expand its reach by adapting its subnet architecture to integrate functions similar to those of protocols like Akash and Gensyn. While Akash provides a decentralized compute marketplace and Gensyn focuses on decentralized model training, Bittensor's flexible design allows it to potentially incorporate these functionalities within its network. By building specialized subnets that handle compute provisioning, model training, and even data marketplace services, Bittensor could emerge as a multi-faceted platform capable of supporting the entire AI development pipeline. But achieving this would require continuous evolution of its incentive models to handle the increased complexity.
Bittensor’s current state reflects its ambitions, as it already hosts subnets achieving remarkable accomplishments in areas like natural language processing, computer vision, and data analysis. With AI models generating millions of data points daily and executing high-throughput processing tasks, the network has demonstrated its potential to handle complex workloads efficiently. The network is still in its early stages, with much of its success relying on the continued engagement of its contributors and the refinement of its economic model. Ensuring the integrity and scalability of its incentive mechanisms will be critical as the network grows and attempts to encompass more varied AI verticals.
The network also aims to refine the TAO tokenomics, ensuring that rewards remain balanced and directly tied to the value of contributions. As the ecosystem expands, the staking mechanisms might evolve to support more nuanced interactions, such as delegating stakes to specific subnets or voting on network upgrades. This dynamic economic model will need to adapt to a growing number of participants and use cases, reinforcing Bittensor’s long-term vision of a decentralized, self-sustaining AI network.
Bittensor’s future involves scaling its subnet ecosystem, enhancing its incentive structures, and exploring new verticals in decentralized AI. Its modular design and collaborative focus position it as a versatile platform that can potentially integrate services similar to those offered by competitors like Akash and Gensyn. While Bittensor has already laid a solid foundation with its unique architecture and network achievements, its success will hinge on its ability to maintain a delicate balance between rewarding high-quality contributions, continuing innovation, and scaling sustainably within the decentralized AI landscape.
Disclaimer: This research report is exactly that — a research report. It is not intended to serve as financial advice, nor should you blindly assume that any of the information is accurate without confirming through your own research. Bitcoin, cryptocurrencies, and other digital assets are incredibly risky and nothing in this report should be considered an endorsement to buy or sell any asset. Never invest more than you are willing to lose and understand the risk that you are taking. Do your own research. All information in this report is for educational purposes only and should not be the basis for any investment decisions that you make.
Disclosure: At least one member of the Reflexivity Research team currently holds a position in Bittensor's token (TAO) at the time of writing/publishing. See the bottom of this write-up for more disclosures.
What is Bittensor?
Bittensor is a decentralized machine learning network built with the aim of creating a global neural network powered by a decentralized, incentive-driven infrastructure. Fundamentally, Bittensor allows AI models to contribute to a shared knowledge pool, receiving cryptocurrency rewards in return for their contributions to the network. The network’s primary goal is to incentivize the creation of intelligent, autonomous AI models by providing a decentralized environment where AI models can interact, learn, and improve in a trust-minimized way.
Bittensor reimagines the traditional model of centralized AI research by introducing decentralized and incentive-based learning. Instead of relying on a few large organizations or companies to provide AI models, Bittensor allows any participant to deploy their own AI model as a "node" in the network. These nodes communicate with each other, exchange information, and use a proof-of-learning mechanism to determine the quality and utility of the knowledge they share. This mechanism creates a competitive environment where the most valuable models receive more rewards, leading to an ecosystem of continuous improvement and innovation.
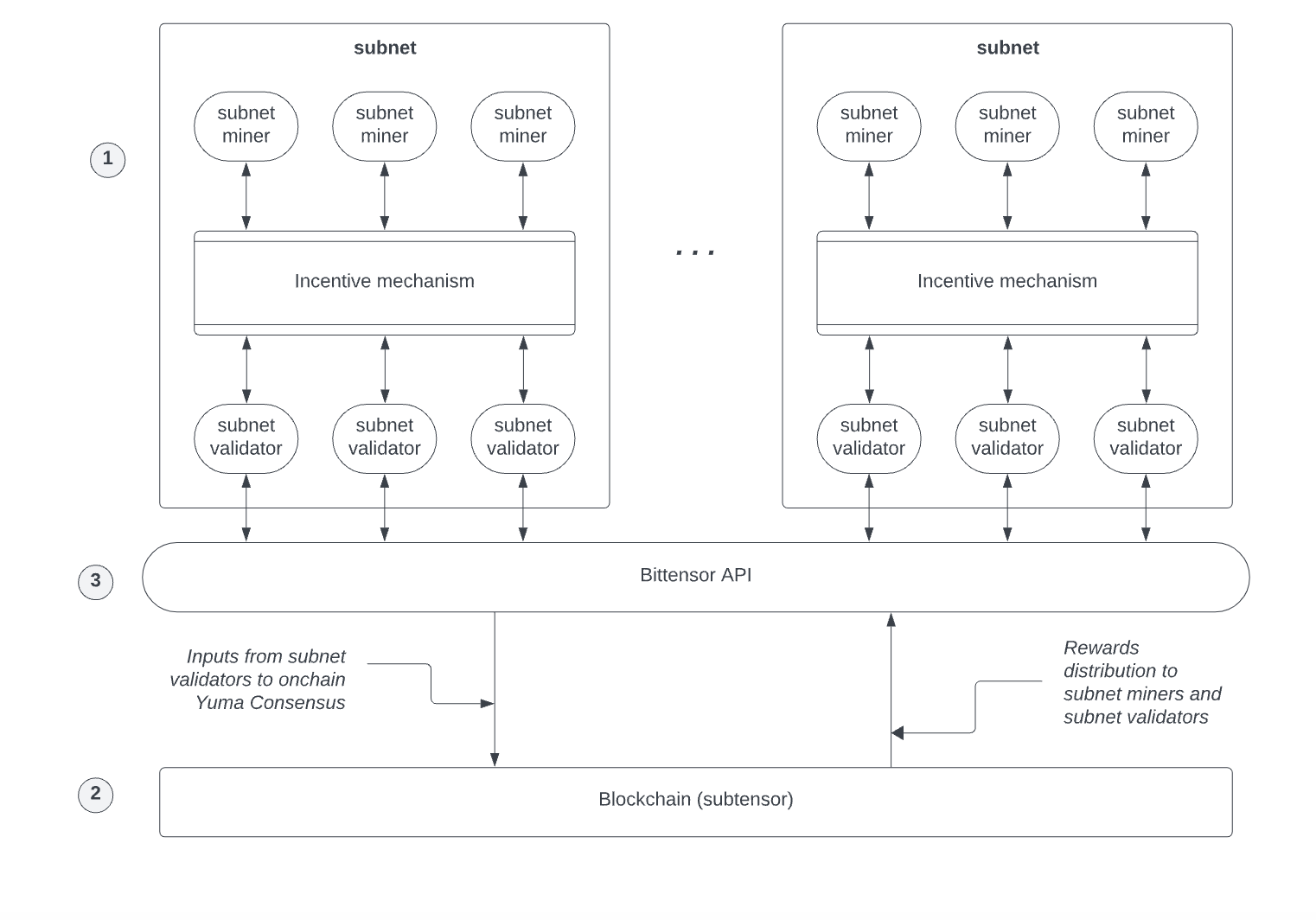
The incentive structure within Bittensor is designed around the network’s native cryptocurrency, TAO. Nodes earn TAO by providing useful information or training data to other nodes. The staking and tokenomics of TAO play a key role in driving the network’s dynamics, as participants stake TAO to access the network’s resources and interact with other nodes. The more valuable a node's contributions, the higher its rewards, thereby creating a self-sustaining cycle of knowledge exchange and refinement.
One of Bittensor’s fundamental goals is to develop an open and permissionless AI network where models of varying capabilities and purposes can collaborate to build more advanced intelligence. The decentralized nature of Bittensor ensures that the knowledge and data shared across the network are not controlled by any single entity, mitigating concerns around data monopolization and crafting a more equitable AI landscape. This contrasts with centralized AI development models, which are often hindered by privacy concerns, limited access to data, and the significant costs associated with model training.
The proof-of-learning mechanism is the heart of Bittensor’s incentive structure. This mechanism assesses the quality of the information provided by each node and its contribution to the overall network intelligence. When a node submits information, other nodes in the network can interact with it, providing feedback on its relevance and accuracy. The more a node's knowledge is utilized and validated by others, the more it earns in TAO. This dynamic encourages nodes to produce high-quality outputs and discourages spam or low-quality contributions, maintaining the integrity of the network.
In addition to the quality-driven incentive model, Bittensor aims to tackle some of the key challenges facing the AI community, such as scalability and interoperability. By creating an open network of interconnected models, Bittensor facilitates the exchange of knowledge across different AI domains, allowing for the rapid development of more versatile AI capabilities. This approach enables the network to evolve organically as new models and technologies are introduced, potentially leading to breakthroughs in AI research that would be more challenging to achieve in isolated, centralized environments.
A key innovation of Bittensor lies in its use of subnets. Bittensor's subnet architecture allows the network to partition nodes into different subgroups, each focusing on specific tasks or datasets. These subnets act as semi-independent neural networks within the larger Bittensor ecosystem, allowing for specialization and efficiency in knowledge sharing. For instance, one subnet might specialize in language processing, while another focuses on image recognition. This compartmentalization not only helps optimize the network's resources but also encourages the development of diverse AI models tailored to specific use cases.
Subnets in Bittensor are designed to be autonomous yet interconnected, enabling nodes within a subnet to communicate and share knowledge relevant to their specialization. Each subnet operates under a shared protocol that defines the rules for interaction, consensus, and reward distribution within that subnet. This structure allows Bittensor to scale effectively by avoiding a one-size-fits-all approach to data processing and knowledge exchange. By dividing the network into focused subnets, Bittensor can handle a broader range of tasks more efficiently, making it a more flexible and powerful platform for AI research and development.
Bittensor’s subnet system introduces a layered approach to the network’s overall intelligence. Within each subnet, nodes work together to improve their specialized knowledge, while still being part of the larger Bittensor network. Nodes in different subnets can cross-communicate, allowing for an exchange of information across different domains of AI. This cross-pollination of knowledge ensures that insights from one subnet can inform and enhance the performance of models in another, creating a dynamic, interconnected web of learning.
One of the key aspects of Bittensor’s subnets is their ability to be permissionless and self-organizing. Any participant can create or join a subnet, and the network dynamically adjusts to the contributions of each node. This open structure fosters a competitive yet collaborative environment, where nodes are incentivized to optimize their contributions to their subnet to maximize their rewards. Additionally, the subnet system allows for rapid experimentation, as developers can launch new subnets to explore novel AI models or training methods without disrupting the entire network.
Each subnet operates under a governance model that is distinct yet aligned with the broader Bittensor network. This model involves consensus mechanisms to validate the contributions of nodes within the subnet and determine the allocation of rewards. These mechanisms ensure that subnets remain efficient and that the knowledge exchanged within them maintains a high standard of quality. Subnet governance also allows for the fine-tuning of the proof-of-learning algorithm to best suit the specific needs and goals of each subnet, enhancing the flexibility and adaptability of the overall network.
The future vision for Bittensor involves decentralizing AI by expanding its network and subnet architecture. The project aims to grow the network's diversity by encouraging the development of new subnets tailored to emerging AI domains, such as natural language processing (NLP), computer vision, reinforcement learning, and more. By increasing the number and variety of subnets, Bittensor hopes to create a vast, decentralized intelligence network where AI models can continually learn, adapt, and evolve through shared knowledge.
Additionally, the TAO token will continue to play a central role in driving the network's growth and participation. Future plans include refining the tokenomics to continue incentivizing high-quality contributions and participation in governance. The staking mechanism might also evolve to support more complex interactions, such as allowing participants to delegate their stake to specific subnets or nodes they believe are most valuable. This will deepen the engagement within the community and enhance the network’s collective intelligence.
Bittensor's roadmap includes expanding its interoperability with other decentralized AI and blockchain projects, facilitating the seamless integration of its knowledge network into broader Web3 ecosystems. This could involve developing bridges to other blockchain platforms, enabling the use of Bittensor’s AI capabilities across decentralized applications (dApps). By positioning itself as a core AI infrastructure within the decentralized world, Bittensor aims to become a key player in shaping the future of AI development.
Bittensor represents a significant leap forward in decentralized AI research. By combining incentivized knowledge sharing, a modular subnet architecture, and a robust proof-of-learning mechanism, it provides a platform where AI models can collaborate, compete, and evolve without the constraints of centralized control. The subnet system enhances the network's scalability and specialization, setting the stage for a new era of AI development driven by decentralized intelligence.
Why is Bittensor necessary?
The current state of artificial intelligence and machine learning is marked by significant centralization, with control and development often limited to large corporations and research institutions. This centralization results in several challenges: the monopolization of valuable datasets, limited access to advanced AI tools for smaller entities, and the potential for bias in model training. Additionally, the closed nature of many AI platforms means that most of the data used for training remains siloed, exacerbating privacy concerns and limiting the diversity of perspectives integrated into AI models. In this landscape, Bittensor presents an alternative approach, leveraging the principles of decentralization and blockchain technology to create an open, incentivized AI network.
Blockchain technology, particularly when combined with decentralized protocols like Bittensor, offers a new paradigm for AI development. Bittensor's network operates under a permissionless framework, allowing anyone to contribute to and benefit from its AI models. This open-access model contrasts sharply with traditional AI research, where access is often restricted to those with extensive resources or institutional support. By incorporating blockchain, Bittensor provides a trust-minimized environment where AI models, or nodes, can interact and exchange knowledge transparently, without relying on a central authority. This decentralized setup is crucial for managing issues such as data privacy, ownership, and access in an equitable manner.
The role of crypto-economics in Bittensor's architecture cannot be overstated. Traditional AI research is usually funded by grants, commercial interests, or internal budgets, which inherently limits the scope and direction of AI projects. In contrast, Bittensor introduces a self-sustaining incentive mechanism powered by its native token, TAO. This system incentivizes nodes to share valuable insights and continuously improve their models. By aligning economic incentives with knowledge sharing, Bittensor creates a collaborative ecosystem where nodes are motivated to contribute high-quality data and intelligence. This is a marked shift from traditional AI, turning research into a competitive yet symbiotic process that ensures continuous model refinement.
A critical aspect of Bittensor's necessity lies in its ability to address the bias and diversity limitations present in current AI models. Centralized AI systems often train on datasets curated by specific entities, leading to potential biases in their outputs. Bittensor, on the other hand, allows nodes worldwide to provide diverse data inputs, contributing to more comprehensive and balanced AI models. This decentralized approach not only democratizes data access but also results in more robust and reliable AI outputs, as the models are continuously validated and improved by a wide range of participants in the network.
Additionally, the network’s proof-of-learning mechanism ensures that rewards are distributed based on the quality of contributions. This framework addresses one of the major issues in AI today: the lack of a standardized, open evaluation process for determining the value of AI outputs. In traditional settings, the quality of AI models is often judged by proprietary benchmarks, limiting the scope for open collaboration. Bittensor's proof-of-learning system offers a transparent, community-driven approach to evaluating contributions, thereby promoting merit-based rewards. This method fosters a more innovative environment, where nodes are encouraged to explore new techniques, optimize their models, and share their findings with the network.
Bittensor’s subnet architecture serves to enhance its sustainability and utility by allowing specialized AI models to collaborate within specific domains. Subnets focus on tasks ranging from natural language processing to computer vision, providing an efficient and dynamic learning environment. In traditional AI development, specialization often occurs in isolated silos, limiting the opportunity for cross-domain knowledge transfer. Bittensor’s interconnected subnets overcome this barrier, creating an ecosystem where different AI models can learn from each other’s advancements. For example, improvements in one subnet’s language processing capabilities can inform models in other subnets, facilitating a holistic growth of network intelligence.
By utilizing blockchain's transparency and security, Bittensor also tackles the issue of data integrity and trust. Traditional AI models often require users to trust the central entity managing the data and the model’s training process, which can lead to concerns over data misuse or manipulation. Bittensor ensures that all transactions and interactions are recorded on a public ledger, allowing participants to verify the authenticity and provenance of the information shared within the network. This transparency builds trust among contributors and end-users, enhancing the network's credibility and long-term viability.
Bittensor’s incentive mechanisms also address a broader issue within the AI and crypto intersection: the need for sustainable funding models that promote continuous innovation. In many AI projects, funding is a one-time effort that relies on grants, investments, or corporate backing, which may lead to short-lived projects with limited development. Bittensor’s tokenized incentive structure provides a continuous stream of rewards, motivating ongoing contributions and improvements. This not only sustains the network but also drives the rapid evolution of AI models, as participants are constantly rewarded for meaningful, high-quality contributions.
Bittensor's use of decentralized AI aligns with the growing demand for AI ethics and data privacy. Traditional AI development often involves the collection and processing of vast amounts of user data, raising concerns about data privacy and misuse. Bittensor’s network mitigates these risks by decentralizing data ownership, allowing contributors to maintain control over their inputs. Additionally, the network's built-in encryption and privacy measures provide a secure environment for data exchange, reinforcing Bittensor's commitment to ethical AI practices.
In the broader context of AI and crypto convergence, Bittensor exemplifies how decentralized AI can revolutionize both industries. It offers a solution to the centralization and exclusivity that currently hinder AI's full potential by providing an open, collaborative platform powered by blockchain and crypto-economic principles. By doing so, Bittensor not only advances AI research but also demonstrates the power of blockchain to enable new models of innovation, equity, and sustainability in the digital economy. This convergence could be a defining factor in the next wave of AI and blockchain applications, positioning Bittensor as a cornerstone of the decentralized intelligence future.
How do Bittensor’s subnets work?
Bittensor's subnet system forms the backbone of its decentralized AI network, allowing for the creation of specialized environments where different AI models can interact, train, and improve autonomously. Unlike traditional machine learning or artificial intelligence development, which often relies on centralized servers and datasets, Bittensor’s subnet architecture introduces a modular and decentralized approach to AI training. This system allows AI models to exist in subnets tailored to specific tasks or datasets, promoting diversity, specialization, and scalability within the network.
Each subnet in Bittensor acts as a semi-independent neural network focused on a particular type of intelligence or data processing. Nodes (AI models) within these subnets work together by exchanging knowledge in the form of input-output pairs, learning from one another, and building a shared understanding of their specific domain. The subnet system is underpinned by Bittensor's proof-of-learning mechanism, which enables nodes to evaluate the quality and relevance of the knowledge shared within the subnet. This fosters a competitive environment where nodes are incentivized to provide valuable, high-quality contributions to earn rewards in TAO tokens.
In traditional AI/ML development, models are trained on static datasets in centralized environments, often requiring extensive computational resources and data privacy considerations. By contrast, Bittensor's subnets offer a dynamic, distributed training environment where nodes can constantly adapt and improve based on real-time interactions with other nodes in the subnet. This decentralized approach addresses several limitations of traditional AI development. For one, it eliminates the need for centralized data ownership, as knowledge is continually shared and validated within the subnets. Additionally, it provides a more scalable method for training models, as the network can easily expand by adding more nodes and subnets, each contributing to the overall intelligence of the ecosystem.
One of the key features of Bittensor’s subnets is their autonomy. Subnets operate independently but remain interconnected with the broader Bittensor network. They function according to specific protocols that dictate how nodes interact, reach consensus, and allocate rewards. This autonomous operation allows each subnet to optimize for its specific tasks, such as natural language processing, image recognition, or reinforcement learning. Despite this autonomy, subnets can still communicate with one another, allowing cross-pollination of knowledge and facilitating a more diverse and robust AI network.
The permissionless nature of Bittensor's subnets is another significant departure from traditional AI models. In centralized AI development, only specific institutions or companies with access to vast resources can contribute to model training and improvement. Bittensor’s subnet system allows anyone to create or join a subnet, regardless of their computational resources. This democratizes AI development and encourages a wider range of contributions, potentially leading to more innovative models and techniques emerging within the network.
The subnet system also introduces a layered approach to Bittensor’s overall intelligence. Each subnet focuses on a specialized task, and nodes within that subnet collaborate to enhance their understanding of their specific domain. The modular design of these subnets means that they can evolve and adapt as the network grows, ensuring that Bittensor remains a cutting-edge platform for decentralized AI. The self-organizing aspect of these subnets means that they can dynamically adjust to changes in participation, knowledge demand, and the overall network environment, maintaining a high level of efficiency and scalability.
Each of these subnets operates independently but contributes to the overall intelligence of the Bittensor network. They are built to handle specific types of data and learning tasks, allowing for specialization that is difficult to achieve in traditional, monolithic AI systems. The cross-communication between subnets enables knowledge gained in one domain to inform others. For example, improvements in natural language processing in Subnet X could enhance the language understanding capabilities of nodes in other subnets, such as those dealing with sentiment analysis in image recognition.
By implementing a proof-of-learning mechanism within each subnet, Bittensor ensures that only high-quality contributions are rewarded, maintaining the integrity and utility of the network. This is a stark contrast to traditional AI systems, where model performance is often dependent on the quality and size of centralized datasets. In Bittensor’s subnets, the decentralized validation process helps to continuously refine and improve the models, promoting a self-sustaining cycle of knowledge sharing and AI evolution.
Exploring the subnet ecosystem
There are over 49 subnets listed on taopill, but this section will focus on a handful recently highlighted by Sami Kassab in an August report. This doesn’t mean every subnet isn’t worth exploring, but rather it’s better to focus on some recent developments for the network and what teams are working to solve.
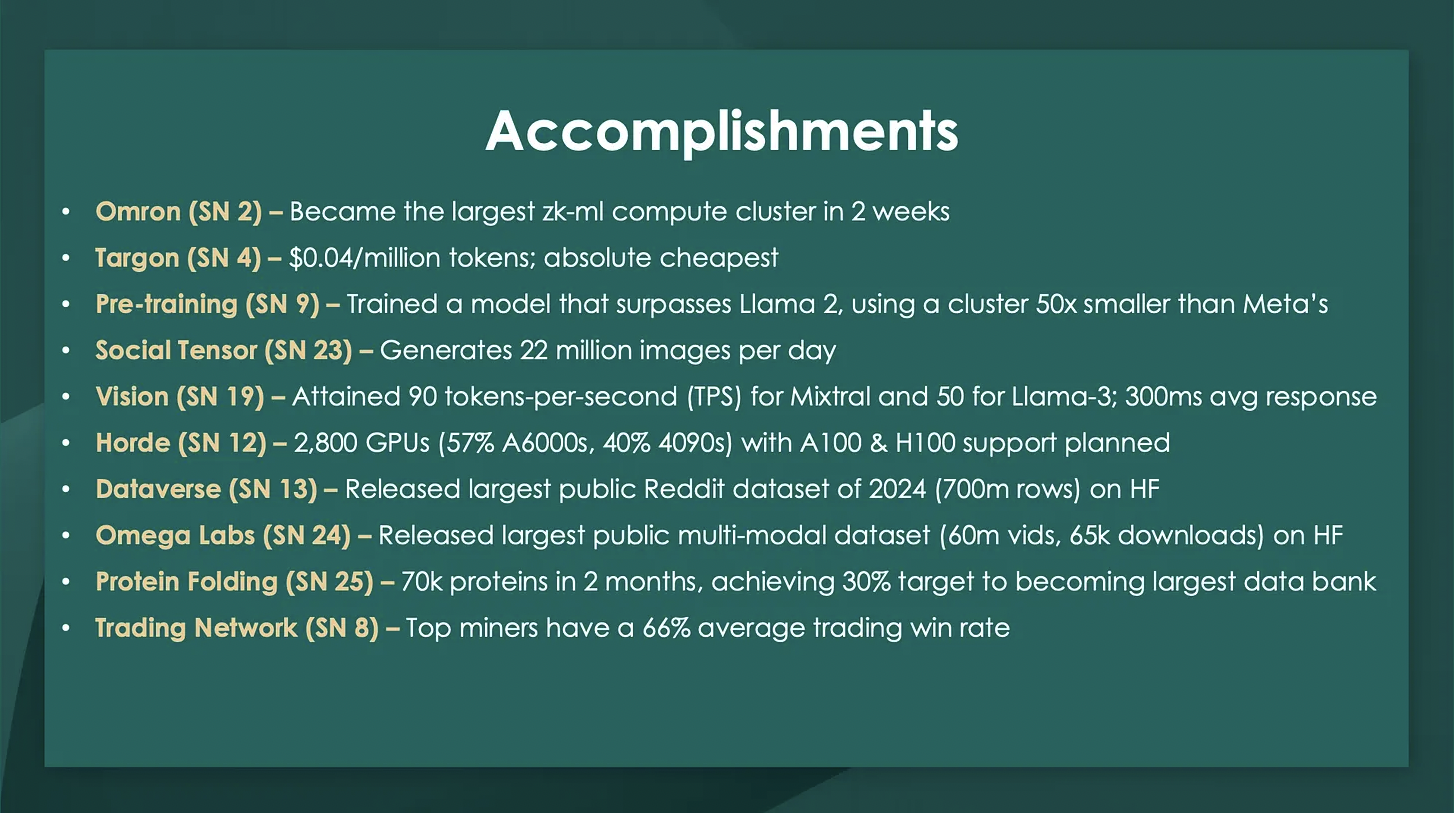
Omron (SN 2) quickly grew to become the largest zero-knowledge machine learning compute cluster in just two weeks. This achievement highlights Bittensor's capability to facilitate rapid development and scaling in decentralized AI.
Zero-knowledge proofs play a critical role in processing data securely without revealing the actual data, addressing privacy concerns often present in centralized AI systems. The success of Omron showcases Bittensor's potential to enable advanced AI solutions while preserving privacy, an essential feature in decentralized networks where data ownership and confidentiality are paramount.
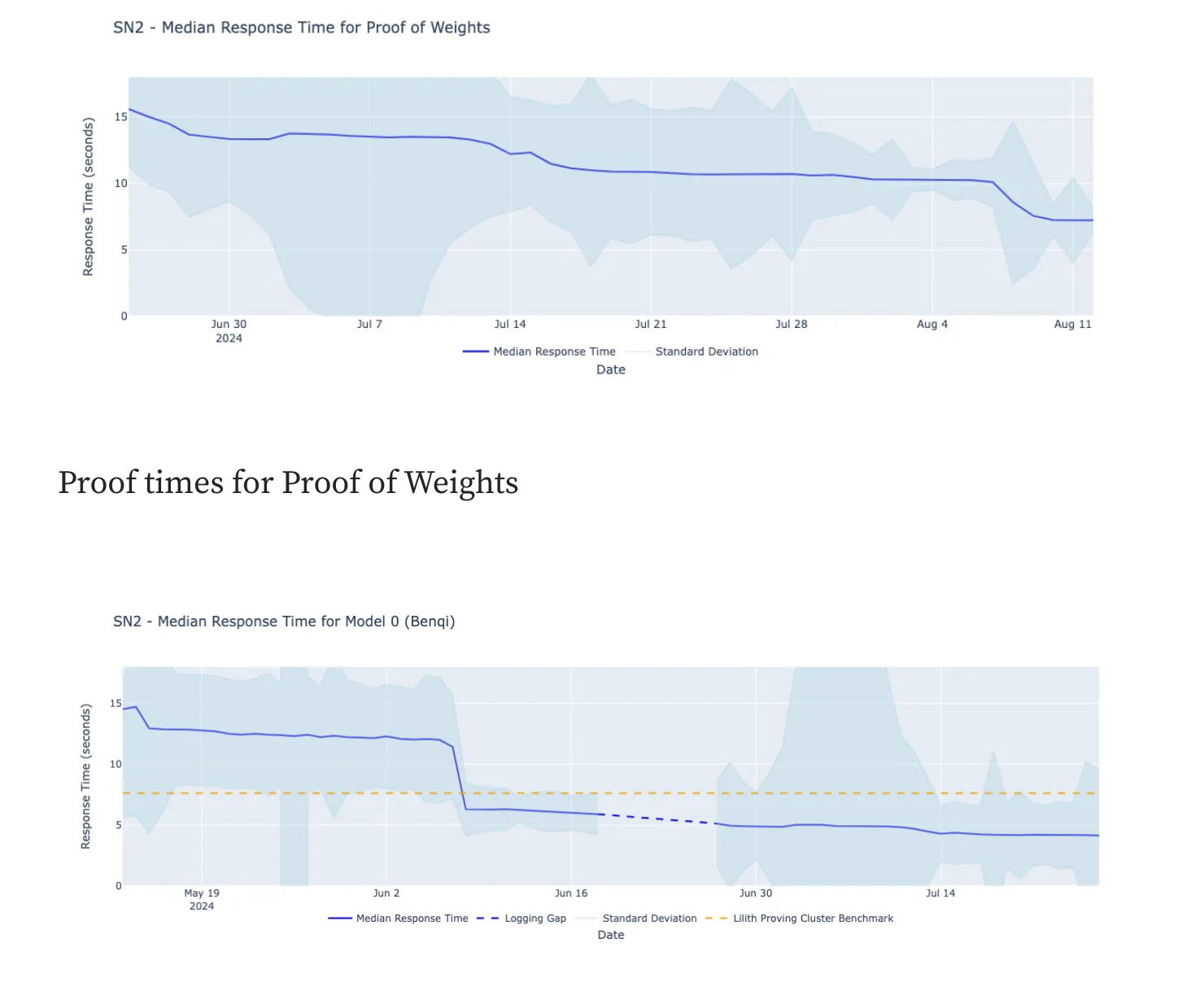
If you’re curious about Omron and want to dig deeper, the team wrote an excellent summary in late August providing their roadmap, current progress, and where they’re headed.
Targon (SN 4) stands out due to its extremely cost-effective computation, charging just $0.04 per million tokens. This approach drastically reduces the economic barriers typically associated with AI research and development, making it accessible to smaller developers, researchers, and independent contributors. In the context of Bittensor, this low-cost model democratizes access to AI capabilities, promoting diversity within the network. By creating an inclusive environment, Targon helps Bittensor attract a broader range of participants, driving the network toward its goal of creating a rich, decentralized AI ecosystem.
Macrocosmos is running a pre-training (SN 9) subnet, and has achieved a remarkable milestone by training a model that surpasses Meta's LLaMA 2, using a compute cluster 50 times smaller than Meta's.
This accomplishment is significant, as it demonstrates that high-performance AI models can be developed in a decentralized network without the need for vast computational resources typical of large tech companies.

By achieving competitive results with a more resource-efficient setup, SN 9 exemplifies how Bittensor's distributed architecture can produce powerful AI solutions. Macrocosmos believes that as time goes on and it becomes increasingly difficult for the average research lab to acquire sufficient amounts of compute, the prospect of decentralized training is only going to further accelerate. This approach aligns with Bittensor's mission to challenge the centralized AI development paradigm and encourage a more open, collaborative landscape for machine learning.
Social Tensor (SN 23) generates an impressive 22 million images daily, positioning itself as a key player in image-based AI models and social media-related tasks. The sheer volume and diversity of images processed within this subnet enrich the network’s capabilities, providing high-quality data for other nodes to leverage. In a decentralized AI ecosystem, access to a wide range of visual data is invaluable for developing models in computer vision, automated content moderation, and real-time image recognition. The output of Social Tensor not only contributes to Bittensor's collective knowledge but also demonstrates how specialized subnets can address complex, data-intensive applications.
Vision (SN 19) showcases Bittensor’s prowess in real-time data processing by achieving 90 tokens-per-second for Mixtral and 50 tokens-per-second for LLaMA-3, with an average response time of just 300ms. This level of performance is crucial for applications requiring immediate feedback, such as autonomous systems, live content filtering, and interactive AI platforms.
By providing rapid and efficient token processing, Vision significantly boosts the network's overall utility, catering to real-world demands for AI that operates in dynamic environments. Its success emphasizes the potential of decentralized AI to handle high-throughput, real-time tasks effectively.
Horde (SN 12) commands an extensive array of 2,800 GPUs, comprising 57% A6000s, 40% A4090s, and additional support from A100 and H100 GPUs. The sheer computational power of this subnet enables it to tackle complex machine learning models that require significant processing resources, such as deep neural networks and advanced reinforcement learning tasks.
Horde's contribution to Bittensor is invaluable, as it lays the foundation for scalability and the capacity to support resource-intensive applications. By pooling computational power from diverse sources, Horde reinforces the network's ability to train sophisticated AI models in a decentralized manner.
Dataverse (SN 13) has released the largest public Reddit dataset of 2024, with 700 million rows accessible on the Hugging Face platform. This dataset serves as a rich repository for NLP and sentiment analysis, enabling nodes within Bittensor to train models capable of understanding and interpreting human language with greater nuance.
In decentralized AI research, publicly available datasets like those provided by Dataverse are vital for incentivizing innovation and model accuracy. By contributing such a comprehensive dataset, Dataverse supports the network's goal of creating open-access AI resources for developers worldwide. For more info on SN 13 and how they’re looking to expand data scraping for Bittensor, their blog is an excellent resource.
Omega Labs (SN 24) brings a multi-modal approach to Bittensor by releasing a dataset containing 60 million videos, with 65,000 downloads on Hugging Face. The diversity and scale of this dataset support the training of models that can process and understand both audio and visual inputs, making it a valuable resource for applications in video analysis, complex audio-visual interactions, and augmented reality.
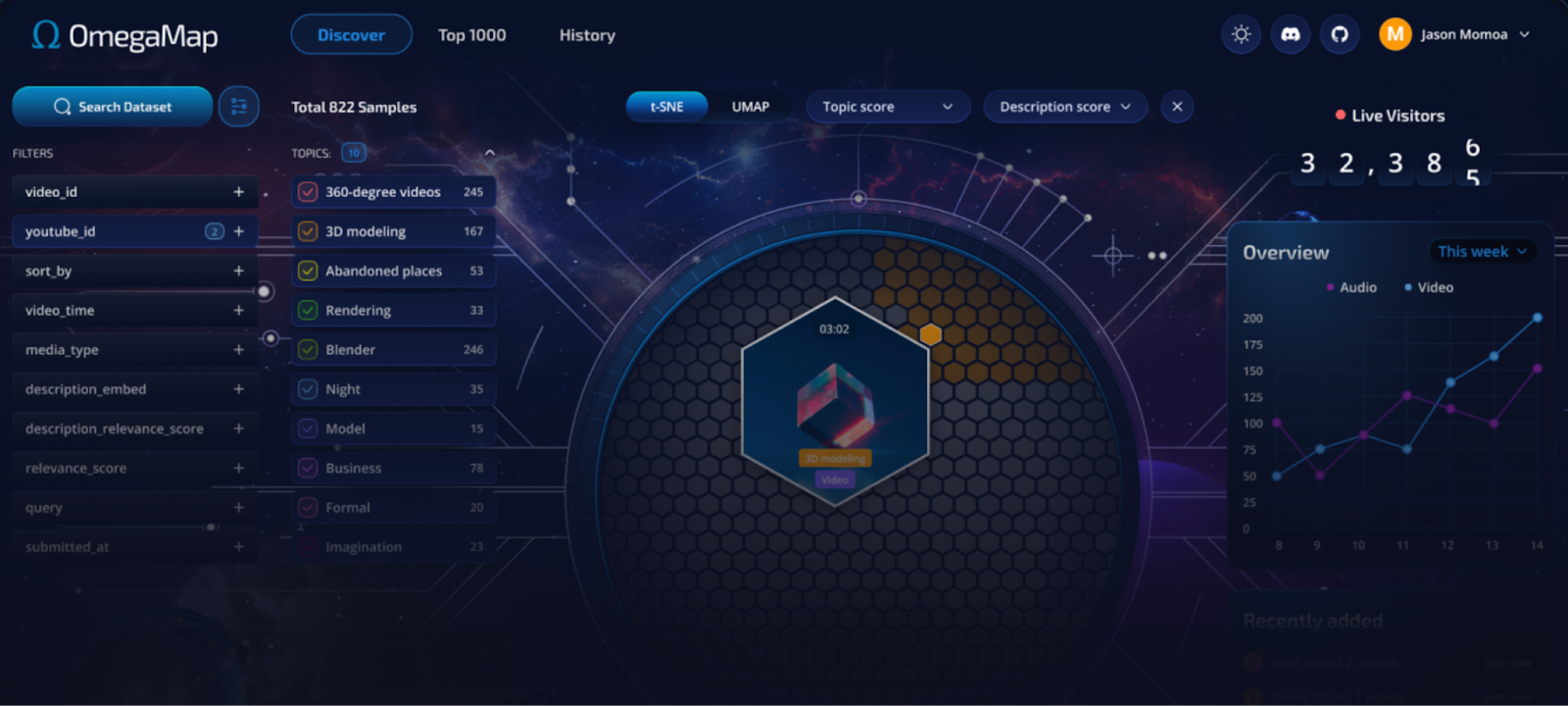
In a decentralized network, multi-modal AI capabilities are critical, as they enable nodes to handle a wide range of data types and use cases. Omega Labs' contribution broadens the scope of Bittensor's ecosystem, facilitating cross-domain learning and enhancing the network's adaptability.
Protein Folding (SN 25) has made significant strides in biological data processing by examining 70,000 proteins in just two months, achieving 30% of its target toward becoming the largest protein data bank in the network. This focus on protein folding has profound implications for biotechnology, pharmaceuticals, and healthcare applications. The team recently posted their plans for scaling SN 25 and what the future of biology on Bittensor might resemble - it’s worth a read.

By providing a decentralized platform for protein analysis, this subnet allows researchers to collaborate on complex biological challenges, potentially accelerating discoveries in drug development and molecular biology. Protein Folding's work illustrates how decentralized AI can impact scientific research domains, leveraging shared computational power and knowledge to solve intricate problems.
Trading Network (SN 8) demonstrates the potential of decentralized AI in financial markets, with top miners achieving an average trading win rate of 66%. This subnet specializes in market analysis and algorithmic trading, utilizing AI models to interpret market trends and execute trades. The success of the Trading Network shows how decentralized intelligence can outperform traditional, centralized trading algorithms, offering more dynamic and responsive market strategies. This subnet not only solidifies Bittensor's relevance in finance but also exemplifies how AI can thrive in decentralized environments, providing valuable insights and strategies in an ever-changing market landscape.
As a whole, these subnets contribute to Bittensor's goal of creating a versatile, scalable AI ecosystem. Their individual successes and focus areas—ranging from natural language processing and computer vision to medical research and financial analysis—enrich the network's collective intelligence.
The diversity of these subnets fosters cross-domain learning, where advancements in one area can inform and enhance models in others. Together, they embody Bittensor's vision of a decentralized, permissionless AI landscape that supports innovation, collaboration, and the continuous evolution of artificial intelligence.
Bittensor and some of its competitors
Bittensor, Akash, and Gensyn are protocols in the decentralized AI and compute space, each approaching different aspects of AI development and deployment. While they target various verticals within the AI and blockchain intersection, they share the common goal of decentralizing computational resources and AI training. However, their strategies, ecosystems, and long-term visions vary significantly, allowing them to coexist while occasionally overlapping in functionality and competition.
Bittensor is primarily focused on building a decentralized, collaborative AI network, emphasizing incentivized knowledge sharing among AI models. What sets Bittensor apart is its ambition to create a global AI network where any participant can contribute to and benefit from the shared pool of intelligence.
By incentivizing high-quality contributions using its native token, TAO, Bittensor has constructed a unique economic model that drives continuous innovation and model refinement within its ecosystem. Its vision goes beyond just training or utilizing AI; it’s about constructing a self-sustaining, decentralized AI society.
On the other hand, Akash Network operates as a decentralized compute marketplace that connects users needing computational resources with providers willing to rent out their unused computing power. It positions itself as a decentralized alternative to traditional cloud providers like AWS and Google Cloud, offering a permissionless and open marketplace for developers to deploy their applications.

Akash does not directly focus on AI or ML but provides the infrastructure necessary for computationally heavy tasks, including AI training. Its strength lies in its flexibility and cost-effectiveness, as users can deploy their projects on an open, peer-to-peer network without being bound to a single provider. This makes Akash a valuable resource for AI projects, potentially including those operating within the Bittensor network, which often require substantial computational resources to train and run models.
Gensyn is more directly aligned with the decentralized AI sector but targets a different aspect: decentralized training. Gensyn offers a protocol designed to facilitate AI model training on a global scale by connecting developers with a decentralized pool of compute resources. It aims to create an environment where AI models can be trained across a distributed network, using cryptographic verification to ensure that training tasks are completed accurately. The team building Gensyn believes that decentralized training is the only path forward - it’s in humanity’s best interest to ensure centralized AI and ML research labs don’t accumulate superintelligence before the rest of us even have a chance to catch up.
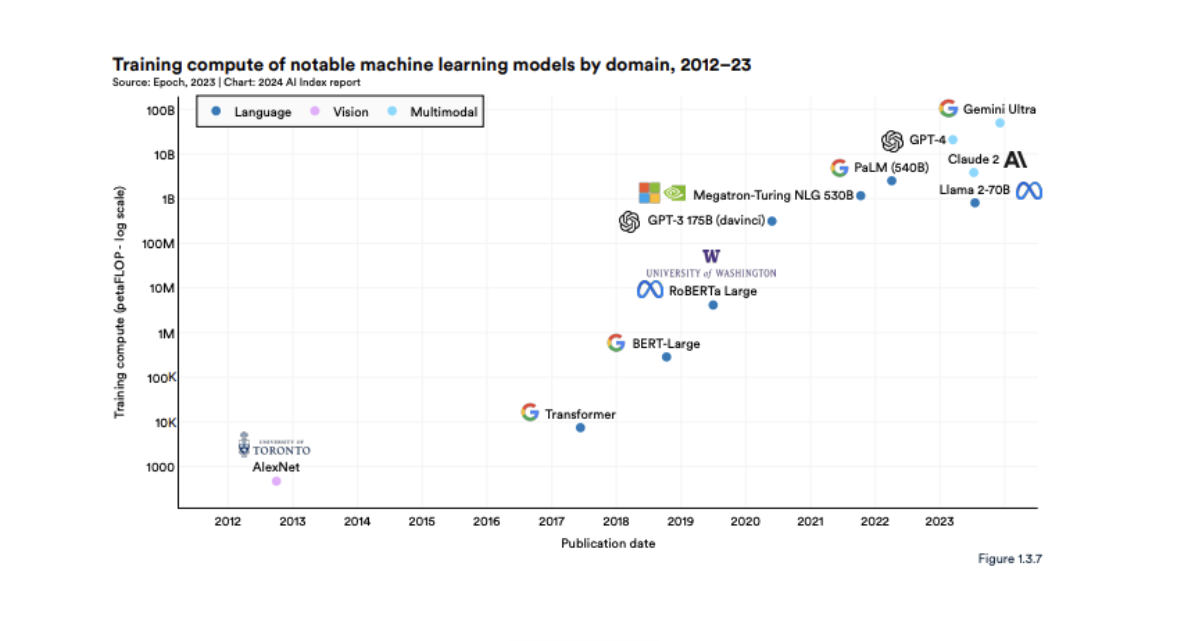
By utilizing blockchain technology to verify and reward contributions to model training, Gensyn focuses on decentralizing one of the most resource-intensive parts of the AI development process: the training phase. Unlike Bittensor, which aims to incentivize both knowledge exchange and model improvement, Gensyn’s primary goal is to create an open marketplace for decentralized model training.
While these three protocols operate in overlapping spaces, their differences in focus create potential for coexistence over longer time horizons. Akash can serve as the computational backbone for projects requiring flexible, decentralized infrastructure, not just for AI but for a broad range of applications. AI-focused projects, including those running on Bittensor or Gensyn, can utilize Akash’s decentralized compute marketplace to access the necessary resources for model training and deployment.
Bittensor and Gensyn, though both operating within the Decentralized AI domain, differ in their major objectives: Bittensor centers on the collaborative exchange of intelligence and the growth of a decentralized AI network, while Gensyn zeroes in on decentralizing AI training, providing developers access to global compute resources to build and refine their models.
Bittensor’s lofty ambitions and unique subnet architecture set it apart, potentially positioning it to compete directly with these protocols in the future. By enabling subnets that can specialize in a variety of tasks, Bittensor can expand into multiple verticals. For instance, subnets focusing on decentralized compute could indirectly compete with Akash by providing an incentive-driven platform for hosting computational workloads, particularly those related to AI. Additionally, Bittensor’s subnet ecosystem could incorporate decentralized model training services akin to Gensyn's, potentially establishing nodes that focus on executing and verifying training tasks within its incentivized framework.
The flexibility of Bittensor’s subnet system means that it could, in theory, integrate aspects of both Akash's compute marketplace and Gensyn’s training verification process into its own network. By doing so, Bittensor could offer a more comprehensive solution, covering the entire lifecycle of AI development, from training and model sharing to deployment and continuous learning. Its collaborative, decentralized nature makes it adaptable to various use cases, allowing it to expand into sectors that Akash and Gensyn currently target. However, for Bittensor to effectively compete in these areas, it would need to maintain the integrity of its incentive mechanisms and ensure that the subnet ecosystem can handle diverse computational tasks efficiently.
While Bittensor, Akash, and Gensyn share common ground in promoting decentralized AI and computational resources, they approach the problem from different angles. Bittensor focuses on building a decentralized AI network that thrives on collaborative intelligence and shared knowledge, leveraging its token-driven incentives to fuel ongoing model evolution. Akash provides the underlying infrastructure for decentralized compute, offering a flexible and cost-effective alternative to centralized cloud providers. Meanwhile, Gensyn zeroes in on the AI training phase, creating a protocol for verifying and rewarding decentralized model training tasks. Together, these protocols contribute to a more open, decentralized AI landscape, with the potential for symbiotic relationships. As Bittensor’s ecosystem evolves, its ability to adapt its subnets to various tasks may allow it to encroach on the domains of both Akash and Gensyn, ultimately competing across multiple verticals in the decentralized AI ecosystem.
Future plans for the network
Bittensor is on the path to becoming a comprehensive, decentralized AI network capable of hosting and evolving a wide variety of AI models through its expansive subnet ecosystem. With its innovative proof-of-learning incentive model and flexible subnet architecture, Bittensor has already set the stage for collaborative, real-time AI development. Moving forward, its primary focus is on scaling this collaborative ecosystem, attracting more participants, and refining its reward mechanisms to ensure that high-quality contributions are consistently incentivized.
One of Bittensor’s goals is to build out its subnet ecosystem, allowing for specialization and the development of models across different AI verticals. By doing so, Bittensor hopes to create a dynamic environment where AI models can evolve and adapt to various tasks, from natural language processing to computer vision and reinforcement learning. The network's permissionless structure will continue to foster inclusivity, attracting developers, researchers, and data scientists who can contribute their expertise and computational resources to grow the network. This approach not only enriches the models within Bittensor but also sets a foundation for tackling more complex and diverse AI challenges in the future.
In terms of competition, Bittensor plans to expand its reach by adapting its subnet architecture to integrate functions similar to those of protocols like Akash and Gensyn. While Akash provides a decentralized compute marketplace and Gensyn focuses on decentralized model training, Bittensor's flexible design allows it to potentially incorporate these functionalities within its network. By building specialized subnets that handle compute provisioning, model training, and even data marketplace services, Bittensor could emerge as a multi-faceted platform capable of supporting the entire AI development pipeline. But achieving this would require continuous evolution of its incentive models to handle the increased complexity.
Bittensor’s current state reflects its ambitions, as it already hosts subnets achieving remarkable accomplishments in areas like natural language processing, computer vision, and data analysis. With AI models generating millions of data points daily and executing high-throughput processing tasks, the network has demonstrated its potential to handle complex workloads efficiently. The network is still in its early stages, with much of its success relying on the continued engagement of its contributors and the refinement of its economic model. Ensuring the integrity and scalability of its incentive mechanisms will be critical as the network grows and attempts to encompass more varied AI verticals.
The network also aims to refine the TAO tokenomics, ensuring that rewards remain balanced and directly tied to the value of contributions. As the ecosystem expands, the staking mechanisms might evolve to support more nuanced interactions, such as delegating stakes to specific subnets or voting on network upgrades. This dynamic economic model will need to adapt to a growing number of participants and use cases, reinforcing Bittensor’s long-term vision of a decentralized, self-sustaining AI network.
Bittensor’s future involves scaling its subnet ecosystem, enhancing its incentive structures, and exploring new verticals in decentralized AI. Its modular design and collaborative focus position it as a versatile platform that can potentially integrate services similar to those offered by competitors like Akash and Gensyn. While Bittensor has already laid a solid foundation with its unique architecture and network achievements, its success will hinge on its ability to maintain a delicate balance between rewarding high-quality contributions, continuing innovation, and scaling sustainably within the decentralized AI landscape.
Disclaimer: This research report is exactly that — a research report. It is not intended to serve as financial advice, nor should you blindly assume that any of the information is accurate without confirming through your own research. Bitcoin, cryptocurrencies, and other digital assets are incredibly risky and nothing in this report should be considered an endorsement to buy or sell any asset. Never invest more than you are willing to lose and understand the risk that you are taking. Do your own research. All information in this report is for educational purposes only and should not be the basis for any investment decisions that you make.
Disclosure: At least one member of the Reflexivity Research team currently holds a position in Bittensor's token (TAO) at the time of writing/publishing. See the bottom of this write-up for more disclosures.
What is Bittensor?
Bittensor is a decentralized machine learning network built with the aim of creating a global neural network powered by a decentralized, incentive-driven infrastructure. Fundamentally, Bittensor allows AI models to contribute to a shared knowledge pool, receiving cryptocurrency rewards in return for their contributions to the network. The network’s primary goal is to incentivize the creation of intelligent, autonomous AI models by providing a decentralized environment where AI models can interact, learn, and improve in a trust-minimized way.
Bittensor reimagines the traditional model of centralized AI research by introducing decentralized and incentive-based learning. Instead of relying on a few large organizations or companies to provide AI models, Bittensor allows any participant to deploy their own AI model as a "node" in the network. These nodes communicate with each other, exchange information, and use a proof-of-learning mechanism to determine the quality and utility of the knowledge they share. This mechanism creates a competitive environment where the most valuable models receive more rewards, leading to an ecosystem of continuous improvement and innovation.
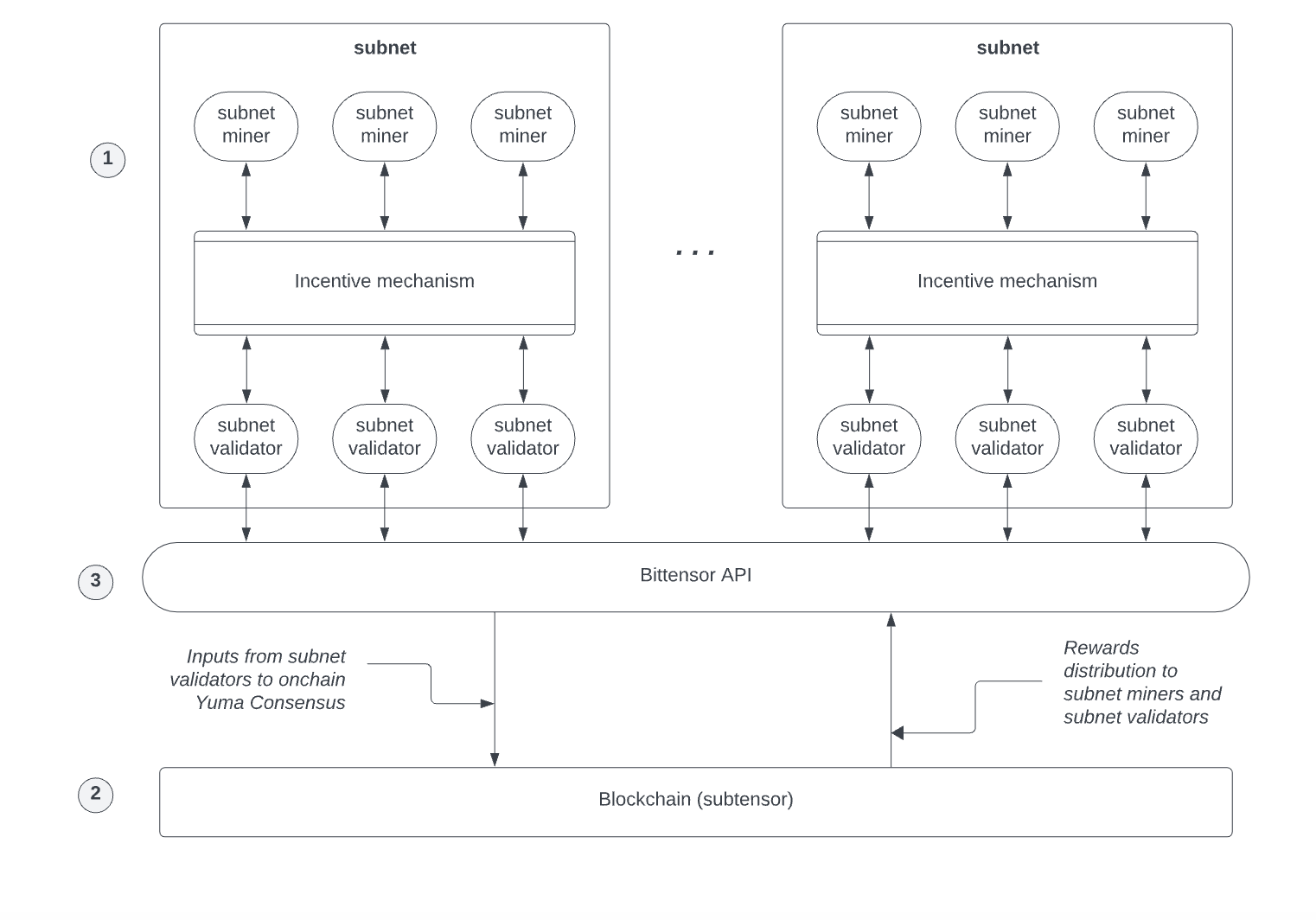
The incentive structure within Bittensor is designed around the network’s native cryptocurrency, TAO. Nodes earn TAO by providing useful information or training data to other nodes. The staking and tokenomics of TAO play a key role in driving the network’s dynamics, as participants stake TAO to access the network’s resources and interact with other nodes. The more valuable a node's contributions, the higher its rewards, thereby creating a self-sustaining cycle of knowledge exchange and refinement.
One of Bittensor’s fundamental goals is to develop an open and permissionless AI network where models of varying capabilities and purposes can collaborate to build more advanced intelligence. The decentralized nature of Bittensor ensures that the knowledge and data shared across the network are not controlled by any single entity, mitigating concerns around data monopolization and crafting a more equitable AI landscape. This contrasts with centralized AI development models, which are often hindered by privacy concerns, limited access to data, and the significant costs associated with model training.
The proof-of-learning mechanism is the heart of Bittensor’s incentive structure. This mechanism assesses the quality of the information provided by each node and its contribution to the overall network intelligence. When a node submits information, other nodes in the network can interact with it, providing feedback on its relevance and accuracy. The more a node's knowledge is utilized and validated by others, the more it earns in TAO. This dynamic encourages nodes to produce high-quality outputs and discourages spam or low-quality contributions, maintaining the integrity of the network.
In addition to the quality-driven incentive model, Bittensor aims to tackle some of the key challenges facing the AI community, such as scalability and interoperability. By creating an open network of interconnected models, Bittensor facilitates the exchange of knowledge across different AI domains, allowing for the rapid development of more versatile AI capabilities. This approach enables the network to evolve organically as new models and technologies are introduced, potentially leading to breakthroughs in AI research that would be more challenging to achieve in isolated, centralized environments.
A key innovation of Bittensor lies in its use of subnets. Bittensor's subnet architecture allows the network to partition nodes into different subgroups, each focusing on specific tasks or datasets. These subnets act as semi-independent neural networks within the larger Bittensor ecosystem, allowing for specialization and efficiency in knowledge sharing. For instance, one subnet might specialize in language processing, while another focuses on image recognition. This compartmentalization not only helps optimize the network's resources but also encourages the development of diverse AI models tailored to specific use cases.
Subnets in Bittensor are designed to be autonomous yet interconnected, enabling nodes within a subnet to communicate and share knowledge relevant to their specialization. Each subnet operates under a shared protocol that defines the rules for interaction, consensus, and reward distribution within that subnet. This structure allows Bittensor to scale effectively by avoiding a one-size-fits-all approach to data processing and knowledge exchange. By dividing the network into focused subnets, Bittensor can handle a broader range of tasks more efficiently, making it a more flexible and powerful platform for AI research and development.
Bittensor’s subnet system introduces a layered approach to the network’s overall intelligence. Within each subnet, nodes work together to improve their specialized knowledge, while still being part of the larger Bittensor network. Nodes in different subnets can cross-communicate, allowing for an exchange of information across different domains of AI. This cross-pollination of knowledge ensures that insights from one subnet can inform and enhance the performance of models in another, creating a dynamic, interconnected web of learning.
One of the key aspects of Bittensor’s subnets is their ability to be permissionless and self-organizing. Any participant can create or join a subnet, and the network dynamically adjusts to the contributions of each node. This open structure fosters a competitive yet collaborative environment, where nodes are incentivized to optimize their contributions to their subnet to maximize their rewards. Additionally, the subnet system allows for rapid experimentation, as developers can launch new subnets to explore novel AI models or training methods without disrupting the entire network.
Each subnet operates under a governance model that is distinct yet aligned with the broader Bittensor network. This model involves consensus mechanisms to validate the contributions of nodes within the subnet and determine the allocation of rewards. These mechanisms ensure that subnets remain efficient and that the knowledge exchanged within them maintains a high standard of quality. Subnet governance also allows for the fine-tuning of the proof-of-learning algorithm to best suit the specific needs and goals of each subnet, enhancing the flexibility and adaptability of the overall network.
The future vision for Bittensor involves decentralizing AI by expanding its network and subnet architecture. The project aims to grow the network's diversity by encouraging the development of new subnets tailored to emerging AI domains, such as natural language processing (NLP), computer vision, reinforcement learning, and more. By increasing the number and variety of subnets, Bittensor hopes to create a vast, decentralized intelligence network where AI models can continually learn, adapt, and evolve through shared knowledge.
Additionally, the TAO token will continue to play a central role in driving the network's growth and participation. Future plans include refining the tokenomics to continue incentivizing high-quality contributions and participation in governance. The staking mechanism might also evolve to support more complex interactions, such as allowing participants to delegate their stake to specific subnets or nodes they believe are most valuable. This will deepen the engagement within the community and enhance the network’s collective intelligence.
Bittensor's roadmap includes expanding its interoperability with other decentralized AI and blockchain projects, facilitating the seamless integration of its knowledge network into broader Web3 ecosystems. This could involve developing bridges to other blockchain platforms, enabling the use of Bittensor’s AI capabilities across decentralized applications (dApps). By positioning itself as a core AI infrastructure within the decentralized world, Bittensor aims to become a key player in shaping the future of AI development.
Bittensor represents a significant leap forward in decentralized AI research. By combining incentivized knowledge sharing, a modular subnet architecture, and a robust proof-of-learning mechanism, it provides a platform where AI models can collaborate, compete, and evolve without the constraints of centralized control. The subnet system enhances the network's scalability and specialization, setting the stage for a new era of AI development driven by decentralized intelligence.
Why is Bittensor necessary?
The current state of artificial intelligence and machine learning is marked by significant centralization, with control and development often limited to large corporations and research institutions. This centralization results in several challenges: the monopolization of valuable datasets, limited access to advanced AI tools for smaller entities, and the potential for bias in model training. Additionally, the closed nature of many AI platforms means that most of the data used for training remains siloed, exacerbating privacy concerns and limiting the diversity of perspectives integrated into AI models. In this landscape, Bittensor presents an alternative approach, leveraging the principles of decentralization and blockchain technology to create an open, incentivized AI network.
Blockchain technology, particularly when combined with decentralized protocols like Bittensor, offers a new paradigm for AI development. Bittensor's network operates under a permissionless framework, allowing anyone to contribute to and benefit from its AI models. This open-access model contrasts sharply with traditional AI research, where access is often restricted to those with extensive resources or institutional support. By incorporating blockchain, Bittensor provides a trust-minimized environment where AI models, or nodes, can interact and exchange knowledge transparently, without relying on a central authority. This decentralized setup is crucial for managing issues such as data privacy, ownership, and access in an equitable manner.
The role of crypto-economics in Bittensor's architecture cannot be overstated. Traditional AI research is usually funded by grants, commercial interests, or internal budgets, which inherently limits the scope and direction of AI projects. In contrast, Bittensor introduces a self-sustaining incentive mechanism powered by its native token, TAO. This system incentivizes nodes to share valuable insights and continuously improve their models. By aligning economic incentives with knowledge sharing, Bittensor creates a collaborative ecosystem where nodes are motivated to contribute high-quality data and intelligence. This is a marked shift from traditional AI, turning research into a competitive yet symbiotic process that ensures continuous model refinement.
A critical aspect of Bittensor's necessity lies in its ability to address the bias and diversity limitations present in current AI models. Centralized AI systems often train on datasets curated by specific entities, leading to potential biases in their outputs. Bittensor, on the other hand, allows nodes worldwide to provide diverse data inputs, contributing to more comprehensive and balanced AI models. This decentralized approach not only democratizes data access but also results in more robust and reliable AI outputs, as the models are continuously validated and improved by a wide range of participants in the network.
Additionally, the network’s proof-of-learning mechanism ensures that rewards are distributed based on the quality of contributions. This framework addresses one of the major issues in AI today: the lack of a standardized, open evaluation process for determining the value of AI outputs. In traditional settings, the quality of AI models is often judged by proprietary benchmarks, limiting the scope for open collaboration. Bittensor's proof-of-learning system offers a transparent, community-driven approach to evaluating contributions, thereby promoting merit-based rewards. This method fosters a more innovative environment, where nodes are encouraged to explore new techniques, optimize their models, and share their findings with the network.
Bittensor’s subnet architecture serves to enhance its sustainability and utility by allowing specialized AI models to collaborate within specific domains. Subnets focus on tasks ranging from natural language processing to computer vision, providing an efficient and dynamic learning environment. In traditional AI development, specialization often occurs in isolated silos, limiting the opportunity for cross-domain knowledge transfer. Bittensor’s interconnected subnets overcome this barrier, creating an ecosystem where different AI models can learn from each other’s advancements. For example, improvements in one subnet’s language processing capabilities can inform models in other subnets, facilitating a holistic growth of network intelligence.
By utilizing blockchain's transparency and security, Bittensor also tackles the issue of data integrity and trust. Traditional AI models often require users to trust the central entity managing the data and the model’s training process, which can lead to concerns over data misuse or manipulation. Bittensor ensures that all transactions and interactions are recorded on a public ledger, allowing participants to verify the authenticity and provenance of the information shared within the network. This transparency builds trust among contributors and end-users, enhancing the network's credibility and long-term viability.
Bittensor’s incentive mechanisms also address a broader issue within the AI and crypto intersection: the need for sustainable funding models that promote continuous innovation. In many AI projects, funding is a one-time effort that relies on grants, investments, or corporate backing, which may lead to short-lived projects with limited development. Bittensor’s tokenized incentive structure provides a continuous stream of rewards, motivating ongoing contributions and improvements. This not only sustains the network but also drives the rapid evolution of AI models, as participants are constantly rewarded for meaningful, high-quality contributions.
Bittensor's use of decentralized AI aligns with the growing demand for AI ethics and data privacy. Traditional AI development often involves the collection and processing of vast amounts of user data, raising concerns about data privacy and misuse. Bittensor’s network mitigates these risks by decentralizing data ownership, allowing contributors to maintain control over their inputs. Additionally, the network's built-in encryption and privacy measures provide a secure environment for data exchange, reinforcing Bittensor's commitment to ethical AI practices.
In the broader context of AI and crypto convergence, Bittensor exemplifies how decentralized AI can revolutionize both industries. It offers a solution to the centralization and exclusivity that currently hinder AI's full potential by providing an open, collaborative platform powered by blockchain and crypto-economic principles. By doing so, Bittensor not only advances AI research but also demonstrates the power of blockchain to enable new models of innovation, equity, and sustainability in the digital economy. This convergence could be a defining factor in the next wave of AI and blockchain applications, positioning Bittensor as a cornerstone of the decentralized intelligence future.
How do Bittensor’s subnets work?
Bittensor's subnet system forms the backbone of its decentralized AI network, allowing for the creation of specialized environments where different AI models can interact, train, and improve autonomously. Unlike traditional machine learning or artificial intelligence development, which often relies on centralized servers and datasets, Bittensor’s subnet architecture introduces a modular and decentralized approach to AI training. This system allows AI models to exist in subnets tailored to specific tasks or datasets, promoting diversity, specialization, and scalability within the network.
Each subnet in Bittensor acts as a semi-independent neural network focused on a particular type of intelligence or data processing. Nodes (AI models) within these subnets work together by exchanging knowledge in the form of input-output pairs, learning from one another, and building a shared understanding of their specific domain. The subnet system is underpinned by Bittensor's proof-of-learning mechanism, which enables nodes to evaluate the quality and relevance of the knowledge shared within the subnet. This fosters a competitive environment where nodes are incentivized to provide valuable, high-quality contributions to earn rewards in TAO tokens.
In traditional AI/ML development, models are trained on static datasets in centralized environments, often requiring extensive computational resources and data privacy considerations. By contrast, Bittensor's subnets offer a dynamic, distributed training environment where nodes can constantly adapt and improve based on real-time interactions with other nodes in the subnet. This decentralized approach addresses several limitations of traditional AI development. For one, it eliminates the need for centralized data ownership, as knowledge is continually shared and validated within the subnets. Additionally, it provides a more scalable method for training models, as the network can easily expand by adding more nodes and subnets, each contributing to the overall intelligence of the ecosystem.
One of the key features of Bittensor’s subnets is their autonomy. Subnets operate independently but remain interconnected with the broader Bittensor network. They function according to specific protocols that dictate how nodes interact, reach consensus, and allocate rewards. This autonomous operation allows each subnet to optimize for its specific tasks, such as natural language processing, image recognition, or reinforcement learning. Despite this autonomy, subnets can still communicate with one another, allowing cross-pollination of knowledge and facilitating a more diverse and robust AI network.
The permissionless nature of Bittensor's subnets is another significant departure from traditional AI models. In centralized AI development, only specific institutions or companies with access to vast resources can contribute to model training and improvement. Bittensor’s subnet system allows anyone to create or join a subnet, regardless of their computational resources. This democratizes AI development and encourages a wider range of contributions, potentially leading to more innovative models and techniques emerging within the network.
The subnet system also introduces a layered approach to Bittensor’s overall intelligence. Each subnet focuses on a specialized task, and nodes within that subnet collaborate to enhance their understanding of their specific domain. The modular design of these subnets means that they can evolve and adapt as the network grows, ensuring that Bittensor remains a cutting-edge platform for decentralized AI. The self-organizing aspect of these subnets means that they can dynamically adjust to changes in participation, knowledge demand, and the overall network environment, maintaining a high level of efficiency and scalability.
Each of these subnets operates independently but contributes to the overall intelligence of the Bittensor network. They are built to handle specific types of data and learning tasks, allowing for specialization that is difficult to achieve in traditional, monolithic AI systems. The cross-communication between subnets enables knowledge gained in one domain to inform others. For example, improvements in natural language processing in Subnet X could enhance the language understanding capabilities of nodes in other subnets, such as those dealing with sentiment analysis in image recognition.
By implementing a proof-of-learning mechanism within each subnet, Bittensor ensures that only high-quality contributions are rewarded, maintaining the integrity and utility of the network. This is a stark contrast to traditional AI systems, where model performance is often dependent on the quality and size of centralized datasets. In Bittensor’s subnets, the decentralized validation process helps to continuously refine and improve the models, promoting a self-sustaining cycle of knowledge sharing and AI evolution.
Exploring the subnet ecosystem
There are over 49 subnets listed on taopill, but this section will focus on a handful recently highlighted by Sami Kassab in an August report. This doesn’t mean every subnet isn’t worth exploring, but rather it’s better to focus on some recent developments for the network and what teams are working to solve.
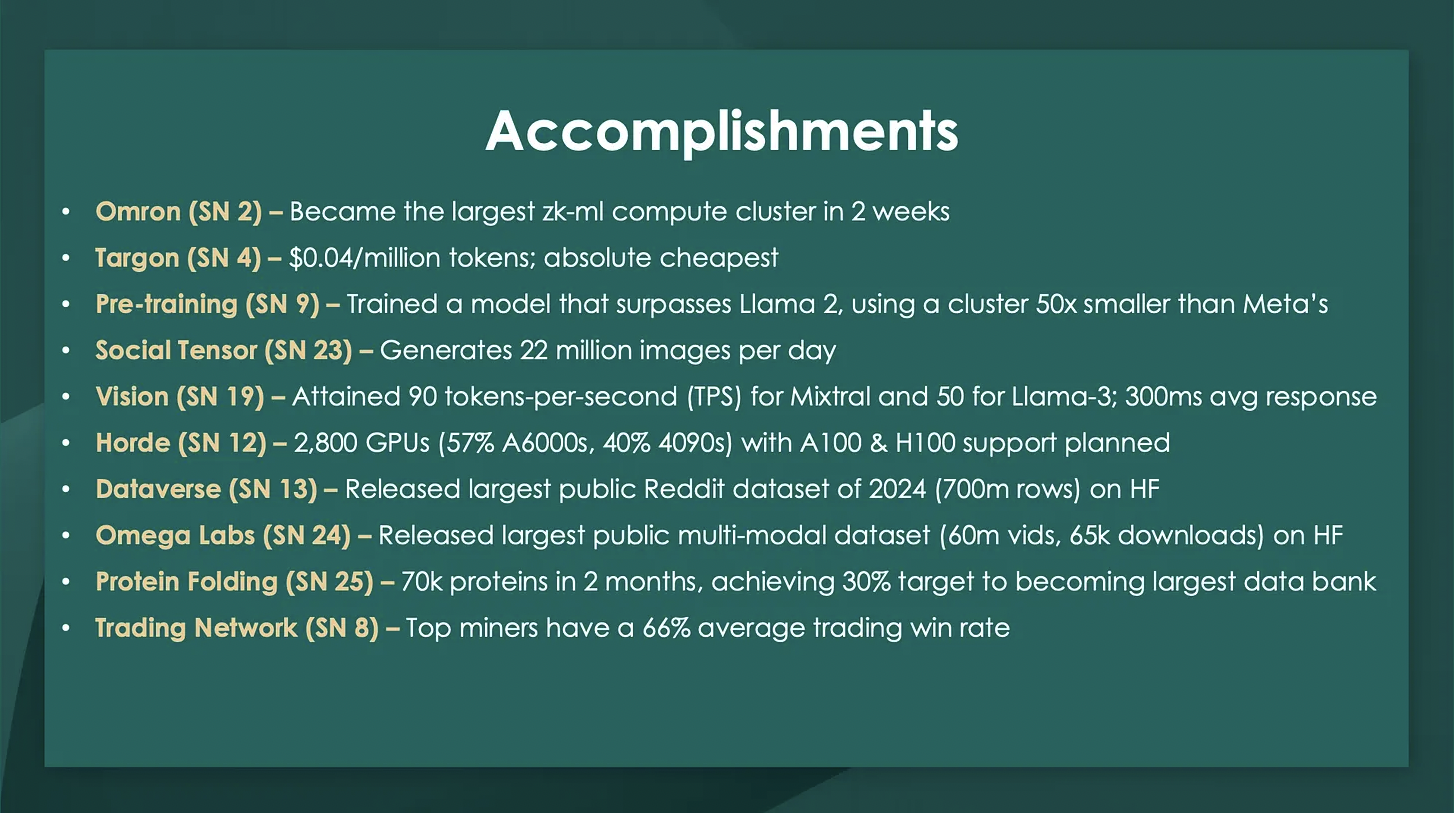
Omron (SN 2) quickly grew to become the largest zero-knowledge machine learning compute cluster in just two weeks. This achievement highlights Bittensor's capability to facilitate rapid development and scaling in decentralized AI.
Zero-knowledge proofs play a critical role in processing data securely without revealing the actual data, addressing privacy concerns often present in centralized AI systems. The success of Omron showcases Bittensor's potential to enable advanced AI solutions while preserving privacy, an essential feature in decentralized networks where data ownership and confidentiality are paramount.
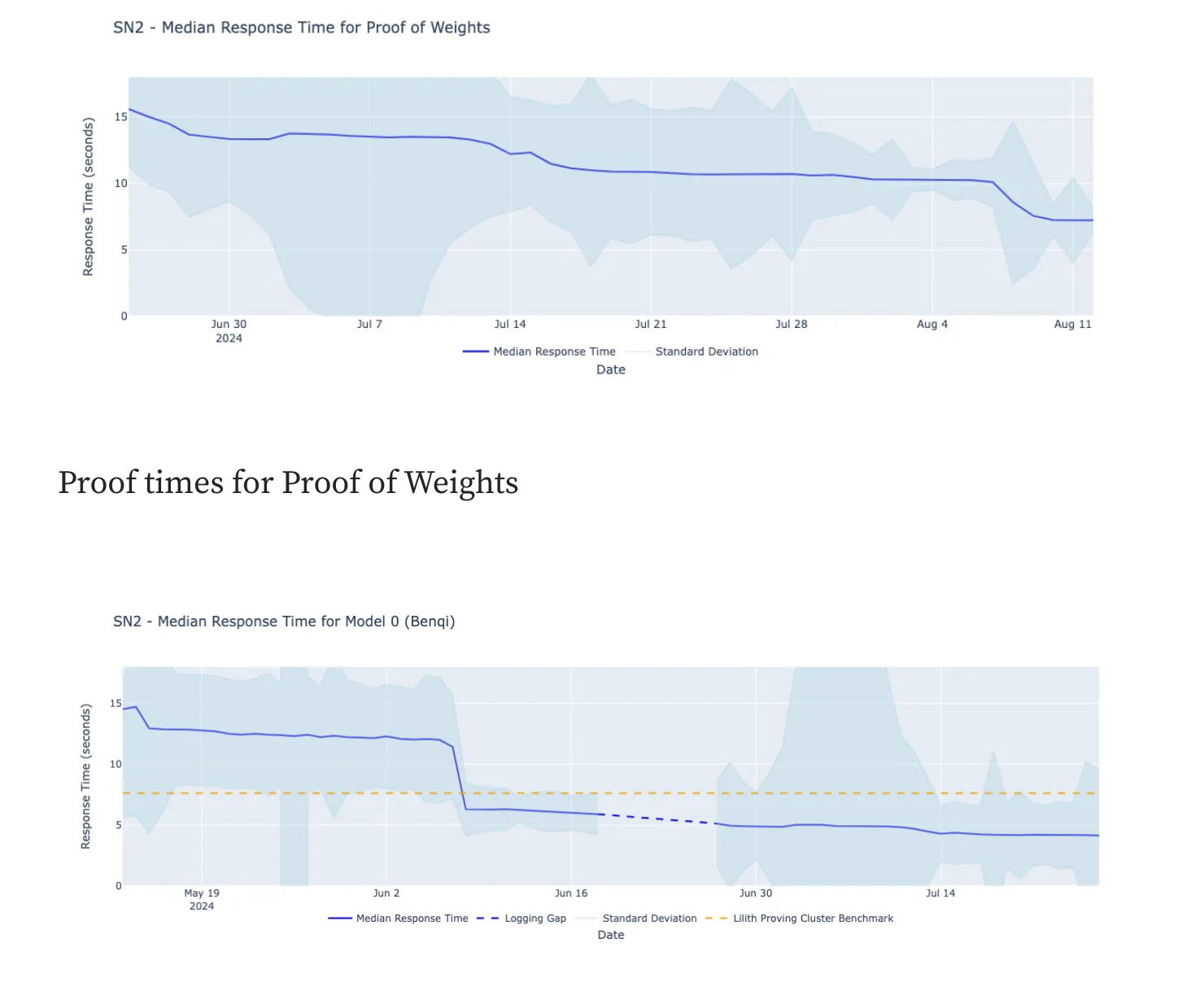
If you’re curious about Omron and want to dig deeper, the team wrote an excellent summary in late August providing their roadmap, current progress, and where they’re headed.
Targon (SN 4) stands out due to its extremely cost-effective computation, charging just $0.04 per million tokens. This approach drastically reduces the economic barriers typically associated with AI research and development, making it accessible to smaller developers, researchers, and independent contributors. In the context of Bittensor, this low-cost model democratizes access to AI capabilities, promoting diversity within the network. By creating an inclusive environment, Targon helps Bittensor attract a broader range of participants, driving the network toward its goal of creating a rich, decentralized AI ecosystem.
Macrocosmos is running a pre-training (SN 9) subnet, and has achieved a remarkable milestone by training a model that surpasses Meta's LLaMA 2, using a compute cluster 50 times smaller than Meta's.
This accomplishment is significant, as it demonstrates that high-performance AI models can be developed in a decentralized network without the need for vast computational resources typical of large tech companies.

By achieving competitive results with a more resource-efficient setup, SN 9 exemplifies how Bittensor's distributed architecture can produce powerful AI solutions. Macrocosmos believes that as time goes on and it becomes increasingly difficult for the average research lab to acquire sufficient amounts of compute, the prospect of decentralized training is only going to further accelerate. This approach aligns with Bittensor's mission to challenge the centralized AI development paradigm and encourage a more open, collaborative landscape for machine learning.
Social Tensor (SN 23) generates an impressive 22 million images daily, positioning itself as a key player in image-based AI models and social media-related tasks. The sheer volume and diversity of images processed within this subnet enrich the network’s capabilities, providing high-quality data for other nodes to leverage. In a decentralized AI ecosystem, access to a wide range of visual data is invaluable for developing models in computer vision, automated content moderation, and real-time image recognition. The output of Social Tensor not only contributes to Bittensor's collective knowledge but also demonstrates how specialized subnets can address complex, data-intensive applications.
Vision (SN 19) showcases Bittensor’s prowess in real-time data processing by achieving 90 tokens-per-second for Mixtral and 50 tokens-per-second for LLaMA-3, with an average response time of just 300ms. This level of performance is crucial for applications requiring immediate feedback, such as autonomous systems, live content filtering, and interactive AI platforms.
By providing rapid and efficient token processing, Vision significantly boosts the network's overall utility, catering to real-world demands for AI that operates in dynamic environments. Its success emphasizes the potential of decentralized AI to handle high-throughput, real-time tasks effectively.
Horde (SN 12) commands an extensive array of 2,800 GPUs, comprising 57% A6000s, 40% A4090s, and additional support from A100 and H100 GPUs. The sheer computational power of this subnet enables it to tackle complex machine learning models that require significant processing resources, such as deep neural networks and advanced reinforcement learning tasks.
Horde's contribution to Bittensor is invaluable, as it lays the foundation for scalability and the capacity to support resource-intensive applications. By pooling computational power from diverse sources, Horde reinforces the network's ability to train sophisticated AI models in a decentralized manner.
Dataverse (SN 13) has released the largest public Reddit dataset of 2024, with 700 million rows accessible on the Hugging Face platform. This dataset serves as a rich repository for NLP and sentiment analysis, enabling nodes within Bittensor to train models capable of understanding and interpreting human language with greater nuance.
In decentralized AI research, publicly available datasets like those provided by Dataverse are vital for incentivizing innovation and model accuracy. By contributing such a comprehensive dataset, Dataverse supports the network's goal of creating open-access AI resources for developers worldwide. For more info on SN 13 and how they’re looking to expand data scraping for Bittensor, their blog is an excellent resource.
Omega Labs (SN 24) brings a multi-modal approach to Bittensor by releasing a dataset containing 60 million videos, with 65,000 downloads on Hugging Face. The diversity and scale of this dataset support the training of models that can process and understand both audio and visual inputs, making it a valuable resource for applications in video analysis, complex audio-visual interactions, and augmented reality.
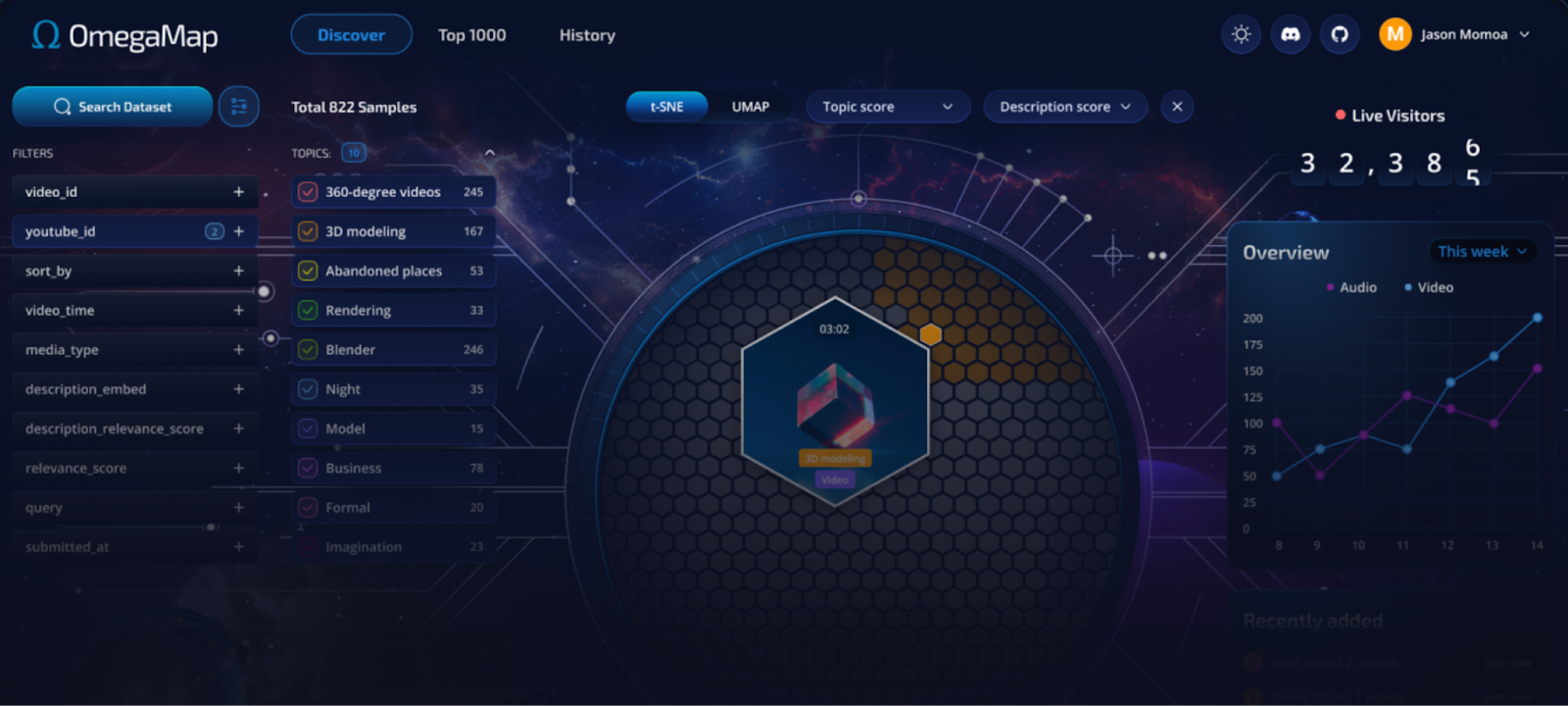
In a decentralized network, multi-modal AI capabilities are critical, as they enable nodes to handle a wide range of data types and use cases. Omega Labs' contribution broadens the scope of Bittensor's ecosystem, facilitating cross-domain learning and enhancing the network's adaptability.
Protein Folding (SN 25) has made significant strides in biological data processing by examining 70,000 proteins in just two months, achieving 30% of its target toward becoming the largest protein data bank in the network. This focus on protein folding has profound implications for biotechnology, pharmaceuticals, and healthcare applications. The team recently posted their plans for scaling SN 25 and what the future of biology on Bittensor might resemble - it’s worth a read.

By providing a decentralized platform for protein analysis, this subnet allows researchers to collaborate on complex biological challenges, potentially accelerating discoveries in drug development and molecular biology. Protein Folding's work illustrates how decentralized AI can impact scientific research domains, leveraging shared computational power and knowledge to solve intricate problems.
Trading Network (SN 8) demonstrates the potential of decentralized AI in financial markets, with top miners achieving an average trading win rate of 66%. This subnet specializes in market analysis and algorithmic trading, utilizing AI models to interpret market trends and execute trades. The success of the Trading Network shows how decentralized intelligence can outperform traditional, centralized trading algorithms, offering more dynamic and responsive market strategies. This subnet not only solidifies Bittensor's relevance in finance but also exemplifies how AI can thrive in decentralized environments, providing valuable insights and strategies in an ever-changing market landscape.
As a whole, these subnets contribute to Bittensor's goal of creating a versatile, scalable AI ecosystem. Their individual successes and focus areas—ranging from natural language processing and computer vision to medical research and financial analysis—enrich the network's collective intelligence.
The diversity of these subnets fosters cross-domain learning, where advancements in one area can inform and enhance models in others. Together, they embody Bittensor's vision of a decentralized, permissionless AI landscape that supports innovation, collaboration, and the continuous evolution of artificial intelligence.
Bittensor and some of its competitors
Bittensor, Akash, and Gensyn are protocols in the decentralized AI and compute space, each approaching different aspects of AI development and deployment. While they target various verticals within the AI and blockchain intersection, they share the common goal of decentralizing computational resources and AI training. However, their strategies, ecosystems, and long-term visions vary significantly, allowing them to coexist while occasionally overlapping in functionality and competition.
Bittensor is primarily focused on building a decentralized, collaborative AI network, emphasizing incentivized knowledge sharing among AI models. What sets Bittensor apart is its ambition to create a global AI network where any participant can contribute to and benefit from the shared pool of intelligence.
By incentivizing high-quality contributions using its native token, TAO, Bittensor has constructed a unique economic model that drives continuous innovation and model refinement within its ecosystem. Its vision goes beyond just training or utilizing AI; it’s about constructing a self-sustaining, decentralized AI society.
On the other hand, Akash Network operates as a decentralized compute marketplace that connects users needing computational resources with providers willing to rent out their unused computing power. It positions itself as a decentralized alternative to traditional cloud providers like AWS and Google Cloud, offering a permissionless and open marketplace for developers to deploy their applications.

Akash does not directly focus on AI or ML but provides the infrastructure necessary for computationally heavy tasks, including AI training. Its strength lies in its flexibility and cost-effectiveness, as users can deploy their projects on an open, peer-to-peer network without being bound to a single provider. This makes Akash a valuable resource for AI projects, potentially including those operating within the Bittensor network, which often require substantial computational resources to train and run models.
Gensyn is more directly aligned with the decentralized AI sector but targets a different aspect: decentralized training. Gensyn offers a protocol designed to facilitate AI model training on a global scale by connecting developers with a decentralized pool of compute resources. It aims to create an environment where AI models can be trained across a distributed network, using cryptographic verification to ensure that training tasks are completed accurately. The team building Gensyn believes that decentralized training is the only path forward - it’s in humanity’s best interest to ensure centralized AI and ML research labs don’t accumulate superintelligence before the rest of us even have a chance to catch up.
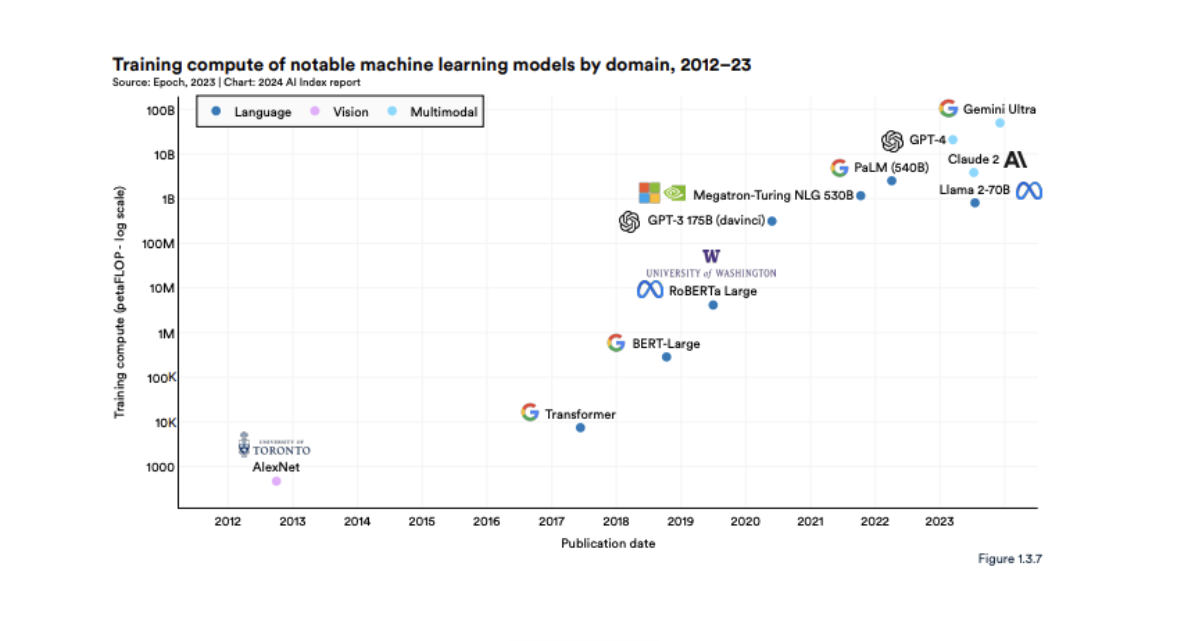
By utilizing blockchain technology to verify and reward contributions to model training, Gensyn focuses on decentralizing one of the most resource-intensive parts of the AI development process: the training phase. Unlike Bittensor, which aims to incentivize both knowledge exchange and model improvement, Gensyn’s primary goal is to create an open marketplace for decentralized model training.
While these three protocols operate in overlapping spaces, their differences in focus create potential for coexistence over longer time horizons. Akash can serve as the computational backbone for projects requiring flexible, decentralized infrastructure, not just for AI but for a broad range of applications. AI-focused projects, including those running on Bittensor or Gensyn, can utilize Akash’s decentralized compute marketplace to access the necessary resources for model training and deployment.
Bittensor and Gensyn, though both operating within the Decentralized AI domain, differ in their major objectives: Bittensor centers on the collaborative exchange of intelligence and the growth of a decentralized AI network, while Gensyn zeroes in on decentralizing AI training, providing developers access to global compute resources to build and refine their models.
Bittensor’s lofty ambitions and unique subnet architecture set it apart, potentially positioning it to compete directly with these protocols in the future. By enabling subnets that can specialize in a variety of tasks, Bittensor can expand into multiple verticals. For instance, subnets focusing on decentralized compute could indirectly compete with Akash by providing an incentive-driven platform for hosting computational workloads, particularly those related to AI. Additionally, Bittensor’s subnet ecosystem could incorporate decentralized model training services akin to Gensyn's, potentially establishing nodes that focus on executing and verifying training tasks within its incentivized framework.
The flexibility of Bittensor’s subnet system means that it could, in theory, integrate aspects of both Akash's compute marketplace and Gensyn’s training verification process into its own network. By doing so, Bittensor could offer a more comprehensive solution, covering the entire lifecycle of AI development, from training and model sharing to deployment and continuous learning. Its collaborative, decentralized nature makes it adaptable to various use cases, allowing it to expand into sectors that Akash and Gensyn currently target. However, for Bittensor to effectively compete in these areas, it would need to maintain the integrity of its incentive mechanisms and ensure that the subnet ecosystem can handle diverse computational tasks efficiently.
While Bittensor, Akash, and Gensyn share common ground in promoting decentralized AI and computational resources, they approach the problem from different angles. Bittensor focuses on building a decentralized AI network that thrives on collaborative intelligence and shared knowledge, leveraging its token-driven incentives to fuel ongoing model evolution. Akash provides the underlying infrastructure for decentralized compute, offering a flexible and cost-effective alternative to centralized cloud providers. Meanwhile, Gensyn zeroes in on the AI training phase, creating a protocol for verifying and rewarding decentralized model training tasks. Together, these protocols contribute to a more open, decentralized AI landscape, with the potential for symbiotic relationships. As Bittensor’s ecosystem evolves, its ability to adapt its subnets to various tasks may allow it to encroach on the domains of both Akash and Gensyn, ultimately competing across multiple verticals in the decentralized AI ecosystem.
Future plans for the network
Bittensor is on the path to becoming a comprehensive, decentralized AI network capable of hosting and evolving a wide variety of AI models through its expansive subnet ecosystem. With its innovative proof-of-learning incentive model and flexible subnet architecture, Bittensor has already set the stage for collaborative, real-time AI development. Moving forward, its primary focus is on scaling this collaborative ecosystem, attracting more participants, and refining its reward mechanisms to ensure that high-quality contributions are consistently incentivized.
One of Bittensor’s goals is to build out its subnet ecosystem, allowing for specialization and the development of models across different AI verticals. By doing so, Bittensor hopes to create a dynamic environment where AI models can evolve and adapt to various tasks, from natural language processing to computer vision and reinforcement learning. The network's permissionless structure will continue to foster inclusivity, attracting developers, researchers, and data scientists who can contribute their expertise and computational resources to grow the network. This approach not only enriches the models within Bittensor but also sets a foundation for tackling more complex and diverse AI challenges in the future.
In terms of competition, Bittensor plans to expand its reach by adapting its subnet architecture to integrate functions similar to those of protocols like Akash and Gensyn. While Akash provides a decentralized compute marketplace and Gensyn focuses on decentralized model training, Bittensor's flexible design allows it to potentially incorporate these functionalities within its network. By building specialized subnets that handle compute provisioning, model training, and even data marketplace services, Bittensor could emerge as a multi-faceted platform capable of supporting the entire AI development pipeline. But achieving this would require continuous evolution of its incentive models to handle the increased complexity.
Bittensor’s current state reflects its ambitions, as it already hosts subnets achieving remarkable accomplishments in areas like natural language processing, computer vision, and data analysis. With AI models generating millions of data points daily and executing high-throughput processing tasks, the network has demonstrated its potential to handle complex workloads efficiently. The network is still in its early stages, with much of its success relying on the continued engagement of its contributors and the refinement of its economic model. Ensuring the integrity and scalability of its incentive mechanisms will be critical as the network grows and attempts to encompass more varied AI verticals.
The network also aims to refine the TAO tokenomics, ensuring that rewards remain balanced and directly tied to the value of contributions. As the ecosystem expands, the staking mechanisms might evolve to support more nuanced interactions, such as delegating stakes to specific subnets or voting on network upgrades. This dynamic economic model will need to adapt to a growing number of participants and use cases, reinforcing Bittensor’s long-term vision of a decentralized, self-sustaining AI network.
Bittensor’s future involves scaling its subnet ecosystem, enhancing its incentive structures, and exploring new verticals in decentralized AI. Its modular design and collaborative focus position it as a versatile platform that can potentially integrate services similar to those offered by competitors like Akash and Gensyn. While Bittensor has already laid a solid foundation with its unique architecture and network achievements, its success will hinge on its ability to maintain a delicate balance between rewarding high-quality contributions, continuing innovation, and scaling sustainably within the decentralized AI landscape.
Disclaimer: This research report is exactly that — a research report. It is not intended to serve as financial advice, nor should you blindly assume that any of the information is accurate without confirming through your own research. Bitcoin, cryptocurrencies, and other digital assets are incredibly risky and nothing in this report should be considered an endorsement to buy or sell any asset. Never invest more than you are willing to lose and understand the risk that you are taking. Do your own research. All information in this report is for educational purposes only and should not be the basis for any investment decisions that you make.